Buzzword technologies are all too familiar within the software engineering world. For years, some of the most creative minds have given us development innovations that have completely changed the landscape of our solutions. On the other hand, some of those trending technologies have caused us much more pain in the long run. All the hype can be difficult to assess.
In this first chapter, I’d like to address the paradigm of reactive programming , which has been gaining popularity for several years, and help to lay the groundwork for our journey into the idea of Reactive Relational Database Connectivity (R2DBC).
Since you’re reading this book, I’m going to assume that you have likely at least heard or read the words “reactive” and “programming” paired together before this point. Heck, I’ll even go out on a limb and guess that you might have even heard or read that in order to create a truly reactive solution, you need to think reactively. But what does all of that even mean? Well, let’s find out!
A Traditional Approach
Introducing new technologies can be challenging, but I’ve found that it can help to identify a common, real-world use case that we, as developers, have encountered and see how it fits within that context.
Imagine a basic solution that contains web request workflow between a client application and a server application, and let’s start at the point where there’s an asynchronous request initiated from the client to the server.
When something is occurring asynchronously, it means that there is a relationship between two or more events/objects that interact within the same system but don’t occur at predetermined intervals and don’t necessarily rely on each other's existence to function. Put more simply, they are events that are not coordinated with each other and happen simultaneously.
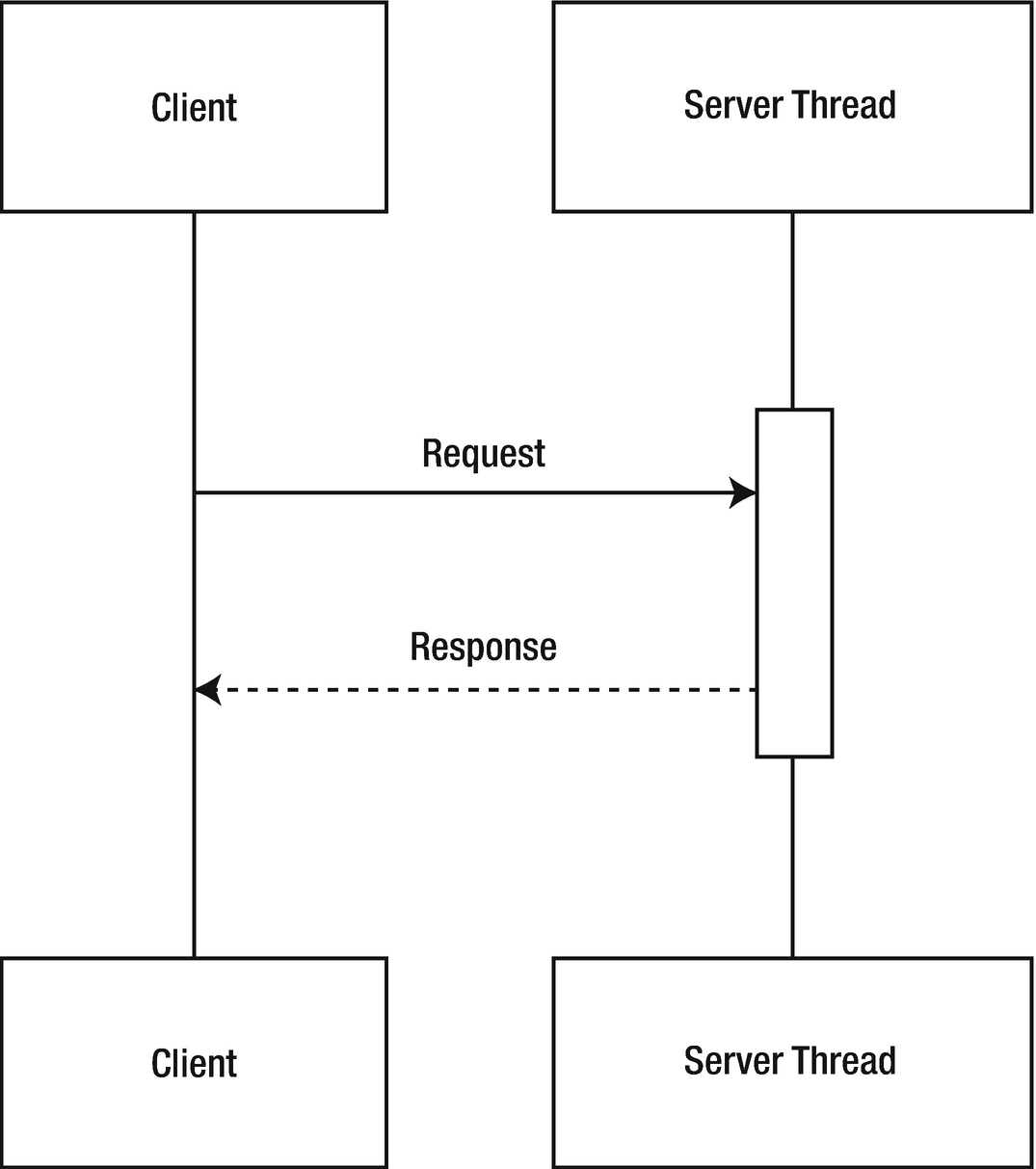
Executing a simple, synchronous web request from a client to server
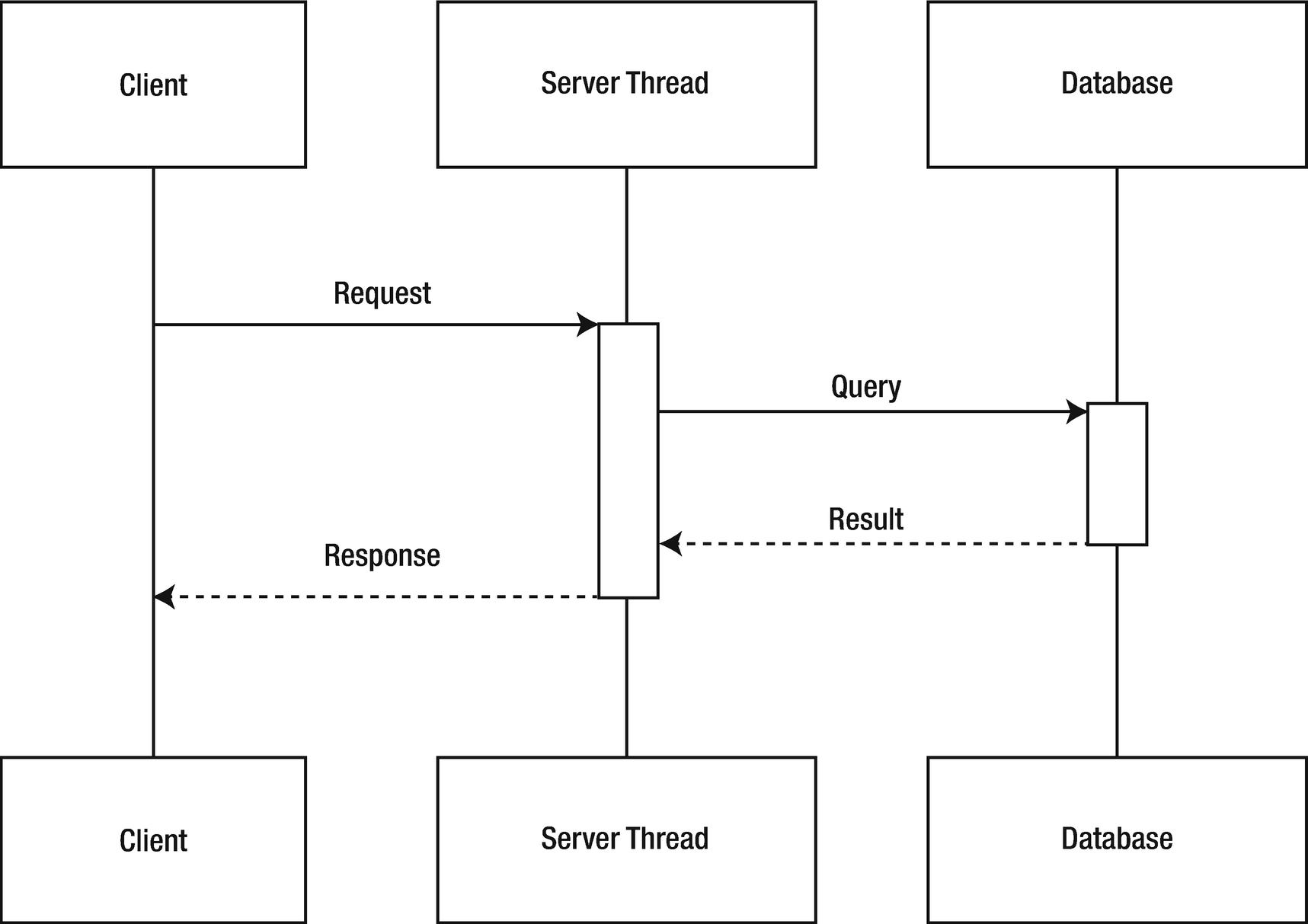
The server thread is blocked from performing work while the database returns a response
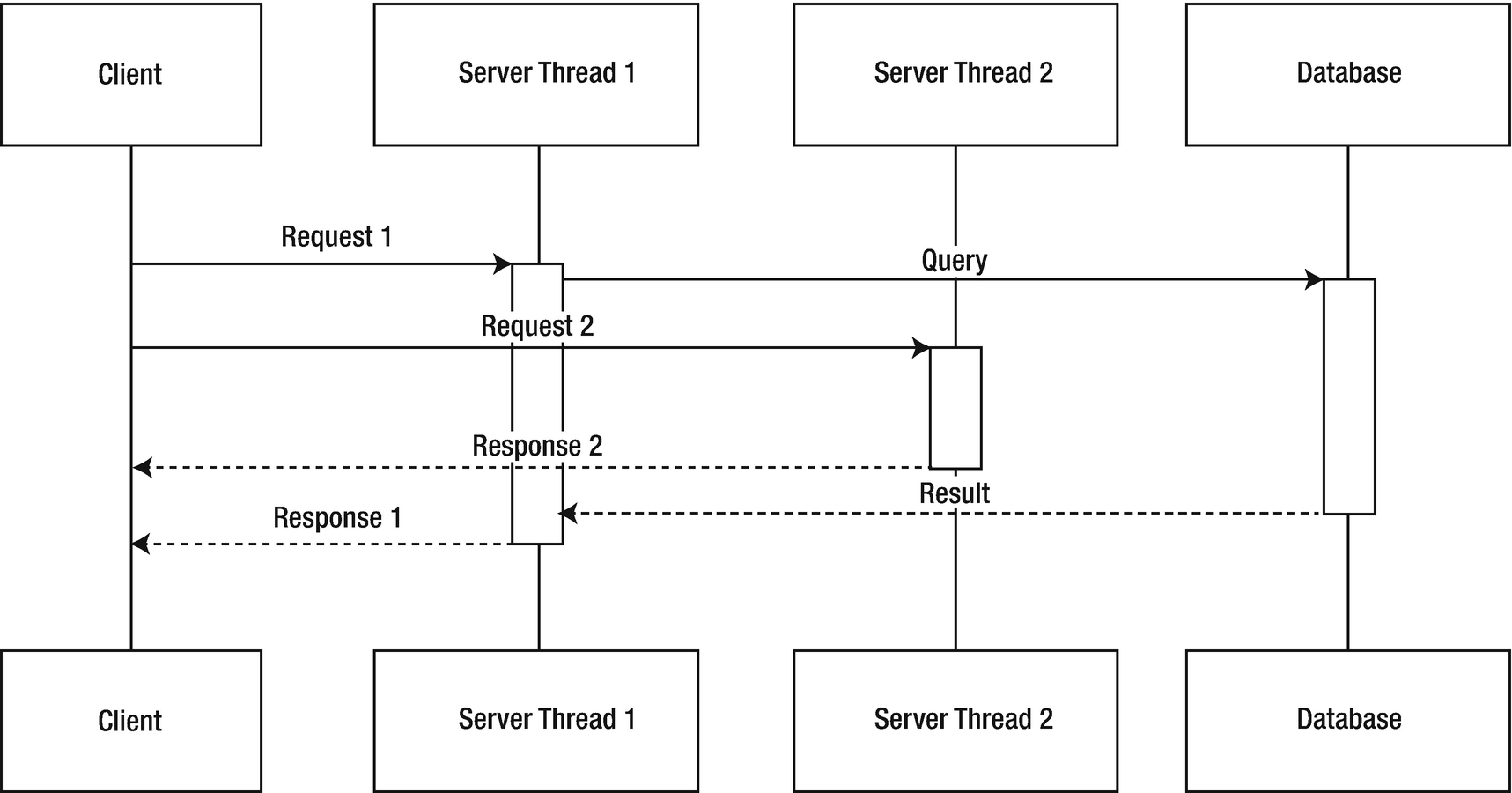
Subsequent incoming requests are processed using additional threads
Now we’re cooking! You can just continue to add threads to handle additional processing, right? Not so fast. As with most things in life, if something seems too good to be true, it probably is. The trade-off for adding additional threads is pesky problems like higher resource consumption, possibly resulting in decreased throughput, and, in many cases, increased complexity for developers due to thread context management.
For many applications, using multiple threads will work as a viable solution. Reactive programming is not a silver bullet. However, reactive solutions can help utilize resources more efficiently. Read on to learn how!
Imperative vs. Declarative Programming
Using multiple threads to prevent blocking operations is a common approach within imperative programming paradigms and languages. That’s because the imperative approach is a process that describes, step-by-step, the state a program should be in to accomplish a specific goal. Ultimately, imperative processes hinge on controlling the flow of information, which can be very useful in certain situations, but can also create quite a headache, as I alluded to before, with memory and thread management.
Declarative programming, on the other hand, does not focus on how to accomplish a specific goal but rather the goal itself. But that’s fairly vague, so let’s back up a bit.
Imperative programming is like attending an art class and listening to the teacher give you step-by-step instructions on how to paint a landscape.
Declarative programming is like attending an art class and being told to paint a landscape. The teacher doesn’t care how you do it, just that it gets done.
Both imperative and declarative programming paradigms have strengths and weaknesses. Like with any task, make sure you choose the right tool for the job!
For this book, I’m going to be using the Java programming languages for all the examples. Now, it’s no secret that Java is an imperative language, and as such its focus is on how to achieve the final result. That said, it’s easy for us to imagine how to write imperative instructions using Java, but what you may not know is that you can also write declarative flows. For instance, consider the following.
In Java 8, streams were introduced as part of an effort to increase the declarative programming capabilities within the language. IntStream rangeClosed(int startInclusive, int endInclusive) returns an IntStream from startInclusive (inclusive) to endInclusive (inclusive) by an incremental step of 1.
However, for the time being, it’s not important that you understand a Stream object or the underlying IntStream specialization. No, for now, set the idea of a “stream” to the side; we’ll get back to it.
The real takeaway here is that both approaches yield the same results, but the declarative approach is merely setting an expectation for the result of an operation, not dictating the underlying implementation steps. This is fundamentally, from a high level, how reactive programming works. Still a little hazy? Let’s dive deeper.
Thinking Reactively
As I mentioned previously, reactive programming, at its core, is declarative. It aims to circumvent blocking states by eliminating many of the issues caused by having to maintain numerous threads. Ultimately, this is accomplished by managing expectations between the client and server.

The client does not wait for a direct response from the server
The non-blocking and event-driven behaviors displayed in Figure 1-4 are the foundation which reactive programming is built on.
The Reactive Manifesto
I know what you’re thinking. The overall concept behind reactive programming isn’t new, so why the hype? Well, it started with the formalization of what constitutes a reactive system.
In 2013, the Reactive Manifesto was created as a way to address new requirements, at the time, in application development and provide a common vocabulary for discussing complex concepts, like reactive programming. And then, in 2014, it was revised, via version 2.0, to more accurately reflect the core value of reactive design.
Reactive Systems
Responsive: The system responds in a timely manner if at all possible. Responsiveness is the cornerstone of usability and utility, but more than that, responsiveness means that problems may be detected quickly and dealt with effectively. Responsive systems focus on providing rapid and consistent response times, establishing reliable upper bounds so they deliver a consistent quality of service. This consistent behaviour in turn simplifies error handling, builds end user confidence, and encourages further interaction.
Resilient: The system stays responsive in the face of failure. This applies not only to highly-available, mission-critical systems—any system that is not resilient will be unresponsive after a failure. Resilience is achieved by replication, containment, isolation and delegation. Failures are contained within each component, isolating components from each other and thereby ensuring that parts of the system can fail and recover without compromising the system as a whole. Recovery of each component is delegated to another (external) component and high-availability is ensured by replication where necessary. The client of a component is not burdened with handling its failures.
Elastic: The system stays responsive under varying workload. Reactive Systems can react to changes in the input rate by increasing or decreasing the resources allocated to service these inputs. This implies designs that have no contention points or central bottlenecks, resulting in the ability to shard or replicate components and distribute inputs among them. Reactive Systems support predictive, as well as Reactive, scaling algorithms by providing relevant live performance measures. They achieve elasticity in a cost-effective way on commodity hardware and software platforms.
- Message Driven: Reactive Systems rely on asynchronous message-passing to establish a boundary between components that ensures loose coupling, isolation and location transparency. This boundary also provides the means to delegate failures as messages. Employing explicit message-passing enables load management, elasticity, and flow control by shaping and monitoring the message queues in the system and applying back-pressure when necessary. Location transparent messaging as a means of communication makes it possible for the management of failure to work with the same constructs and semantics across a cluster or within a single host. Non-blocking communication allows recipients to only consume resources while active, leading to less system overhead.Figure 1-5
The four tenets of the Reactive Manifesto
Put more simply, a reactive system is an architectural approach that seeks to combine multiple, independent solutions into a single, cohesive unit that, as a whole, remains responsive, or reactive, to the world around it with the solutions simultaneously remaining aware of each other. And put as simply as possible, a reactive system is when a unit, adhering to a set of guidelines, of the system remains reactive to each of the other units within the same system that, using those same guidelines, is collectively reactive to external systems.
Reactive Systems != Reactive Programming
At this point, it would be easy to confuse the terms “reactive system” and “reactive programming” as being interchangeable, but it’s important to note that the use of reactive programming within a solution does not make the solution a reactive system.
As previously mentioned, the Reactive Manifesto was revised a year after it was created, and one of the updates was to establish one of the core tenants of reactive systems to involve the usage of asynchronous message-passing . Reactive programming, on the other hand, is event-driven .

Reactive systems vs. reactive programming
An event is a signal by one component upon reaching a certain state that can be observed by any attached listeners. Don’t worry. I’ll dive deeper into how events are observed by listeners in the upcoming sections.
Asynchronous Data Streams
But working with events isn’t a novel concept. In fact, user interface events, like button clicks and various other control interactions, are nothing more than asynchronous event streams that can be subscribed to, observed, and reacted to.
Data Streams
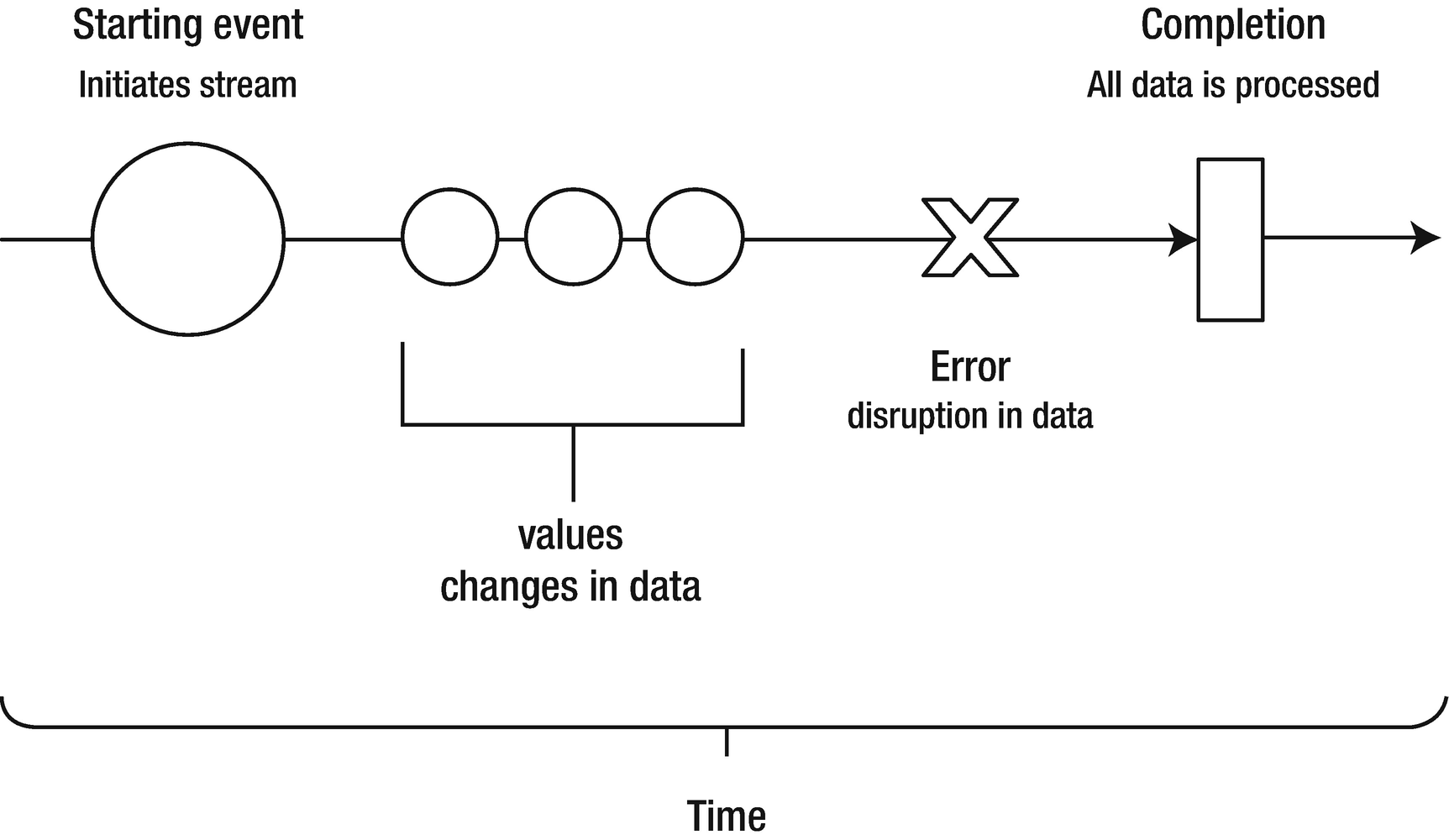
The anatomy of a data stream

Subscribing observers respond to emitted events from the data stream
At this point, it’s important to note that data streams are not limited to sending only user interface events. In fact, reactive programming as a whole wouldn’t be very interesting or so broadly useful if that were the case. So, just as the name suggests, if it’s data, which most things are, it can be streamed in a data stream. This includes, but is certainly not limited to, variables, user input values, properties, objects, and data structures.
If this all seems somewhat familiar, it’s likely because you, at some point in your life, have learned about the Observer Design Pattern .
The Observer Design Pattern is defined as a one-to-many relationship between objects such as if one object is modified, its dependent objects are to be notified automatically.
Back Pressure

The ideal state of a publisher and subscriber
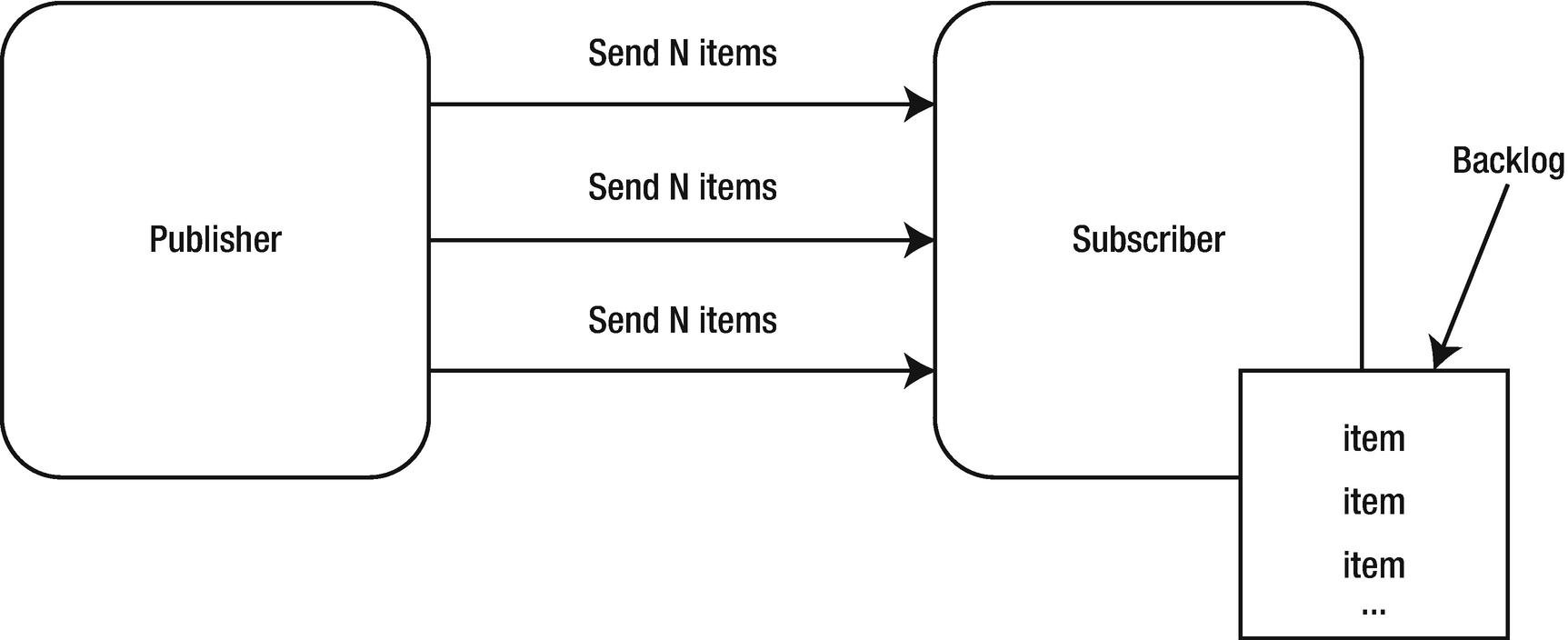
If the publisher emits elements faster than the subscriber can handle them, a backlog of unprocessed elements is created

The simplest implementation of back pressure
Using back pressure to efficiently utilize asynchronous data streams plays a pivotal role in facilitating efficient reactive programming solutions. However, it’s also not a trivial problem to solve, but, luckily, there are a variety of methods through which back pressure can be implemented. In the next chapter, I’ll explore a specification called Reactive Streams that R2DBC uses to create truly reactive database communication.
Summary
In this chapter, you received an overview of what reactive programming is, when it’s useful, and how it works. You’ve gained a high-level understanding of how declarative programming helps facilitate asynchronous data streams to create non-blocking, reactive solutions.
In the next chapter, we’ll examine how these principles have been utilized to facilitate reactive interactions with relational databases using Reactive Relational Database Connectivity (R2DBC).