Adaptation for growth as a common goal throughout the lifespan: Why and how
Rachel Wua,*; Carla Strickland-Hughesb a Department of Psychology, UC Riverside, Riverside, CA, United States
b Psychology Department, University of the Pacific, Stockton, CA, United States
* Corresponding author: email address: [email protected]
Abstract
Thriving in a constantly changing environment requires human learners to adapt. In turn, adaptation requires learning new information and skills (i.e., adaptation for growth). Although specific personal goals change across the lifespan, the need for adaptation for growth is common across all ages. Yet, research with older adults often focuses on adaptation to loss, whereas research with infants and children often focuses on adaptation for growth. However, recent aging research demonstrates the possibility of cognitive maintenance, or even growth, in older adulthood. Focusing more on gains rather than losses may lead to a better understanding of adaptation for growth, and ultimately functional independence in a dynamic environment. After briefly reviewing theories on cognitive growth across the lifespan, we present a novel theoretical framework to explain why and how human learners adapt to grow in a dynamic environment from infancy to older adulthood. This framework highlights the role of real-world skill learning on three intermediate elements of learning to adapt relevant for any age. A driving metaphor conceptualizes these three elements: (1) learning what to learn and how to learn (GPS), (2) motivation to learn and adapt (fuel), and (3) cognitive abilities for learning (engine). We propose that these three elements lead to functional independence in a dynamic environment. We explain how the new framework builds on and extends existing learning research with older adults. Implications and future directions to raise the standard for cognitive aging from loss prevention and maintenance to adaptation for cognitive growth are discussed.
Keywords
Cognitive development; Cognitive aging; Learning; Adaptation; Motivation
1 Introduction
Imagine you have a neighbor named Nicolas. He is in his late 70s, and he is struggling to learn English because he just immigrated to the United States. He has difficulty remembering where he left his favorite book, and he watches television all day. He also does not really talk to anyone. What would you do in this situation as Nicolas' neighbor?
When we pose this question to audiences in academic and non-academic talks, most people say that they would try to have lunch or dinner with Nicolas once in a while to provide some social contact. Then the room typically goes silent, devoid of other suggestions. We then ask, what if Nicolas were a 4-year-old who just immigrated? The room typically emits a murmur of embarrassment as they realize the age-based double standard (Erber & Rager, 1999). For 4-year-old Nicolas, they would encourage his parents to enroll him in enrichment and therapy programs, hire speech tutors, and utilize other assistance programs in the community to help young Nicolas learn and grow.
So why does an age-based double standard exist at opposite ends of the lifespan? It may be because some people still assume that there should be growth earlier in the lifespan and little growth, if any, later in the lifespan. These views follow the outdated, pessimistic view on cognitive aging: that older adulthood (typically 65 + years of age) is a period of universal, biologically-driven, and irreversible decline across all domains (Botwinick, 1967). Following a paradigm-shifting theory that there can be both gains and losses in older adulthood (Baltes, 1987, 1997), recent research on successful aging (e.g., Depp & Jeste, 2006), cognitive enrichment and plasticity (e.g., Hertzog, Kramer, Wilson, & Lindenberger, 2008; Lövdén, Wenger, Martensson, Lindenberger, & Bäckman, 2013; Wilson, Scherr, Schneider, Tang, & Bennett, 2007), and “superagers” or “supernormals” who have higher than normal memory capacity (e.g., Lin, Wang, Wu, Rebok, & Chapman, 2017) demonstrate that the process of cognitive aging is heterogeneous, and one's activities may modify unfavorable cognitive trajectories. We summarize recent findings on cognitive gains in aging in this paper. There is also strong scientific and anecdotal evidence of learning new information and skills in older adulthood, such as older adults embarking on “encore” careers after retiring from another career (Quinn, 2010) or learning a new sport (e.g., Marzorati, 2016). Yet, the age-based double standards persist.
Why is it important to eliminate any age-based double standard to promote growth during adulthood? First, human development should be considered within the environmental context (e.g., Bronfenbrenner, 1994; Gerstof & Ram, 2012; Golant, 2015; Wahl & Gerstorf, 2018). The environment is dynamic. In this constantly changing environment, human learners need to adapt to thrive, and adaptation requires learning new information and skills (i.e., adaptation for growth; Nguyen, Leanos, Natsuaki, Rebok, & Wu, 2018). Therefore, adaptation for growth is a common goal, regardless of one's life stage.
In what ways is the environment dynamic and demanding? An obvious, but rapid and significant, way in which the environment changes is via technological advances (e.g., Charness & Boot, 2009, 2016). For example, medical records are becoming accessible only online. Without learning a new online platform, patients will become dependent on others to access their private medical records. In addition, as cell phone providers disconnect 3G networks, users also need to upgrade their phones, in many cases to smartphones. Similarly, rideshare apps, such as Uber and Lyft, provide a convenient way of navigating and maintaining independence, especially in a foreign city or for those who can no longer drive themselves, but only if one has the apps on a smartphone. Therefore, what might seem as a convenience at first (e.g., online banking, online medical records) may become necessary within a few years. Beyond technological advances, the environment may change due to social role transitions, such as the first day of school, first child, or first day of retirement. Environmental changes also may result from societal changes, such as new laws and policies or urbanization/gentrification. Whenever there are changes in the social or physical environment, learners have to decide whether to adapt and learn something new or not adapt or learn, which may lead to barriers in future social or environmental circumstances (see also Baltes & Baltes, 1990). The more that changes, the more learning decisions have to be made.
By drawing on research with infants and children focusing on adaptation for growth, this paper aims to build on and extend recent aging research demonstrating the possibility of cognitive maintenance, or even growth, in older adulthood. A better understanding of adaptation for growth in adulthood may provide a new perspective on maintaining functional independence in a dynamic environment. This paper first briefly reviews theories on cognitive growth in infants and children. Next, we present a novel theoretical framework to explain why and how human learners adapt to grow in a dynamic environment from infancy to older adulthood. This framework highlights the role of real-world skill learning on three intermediate elements of learning to adapt relevant for any age: the GPS, fuel, and engine that drive adaptation for growth. First, the GPS is learning what to learn and how to learn. Second, the fuel is motivation to learn and adapt, and, third, the engine is cognitive abilities for learning. We propose that these three elements lead to functional independence in a dynamic environment. We explain how the new framework builds on and extends existing learning research with older adults. Finally, we discuss implications and future directions to raise the standard for cognitive aging from loss prevention and maintenance to adaptation for cognitive growth.
2 Learning and cognitive growth in infancy and childhood
For the past few decades, child cognitive development research has garnered a great deal of knowledge on various learning mechanisms and optimal learning environments in different contexts. For example, studies have focused on how infants and children reduce the problem space by learning what to learn (for a review, see Wu, 2019), or when and how they use particular learning mechanisms, such as statistical learning, to acquire language and object understanding (e.g., Aslin & Newport, 2012). Theoretical approaches in child development encourage making mistakes to maximize learning (e.g., growth mindset; Dweck, 2006) and help the child advance to the next higher level in learning (i.e., scaffolding, zone of proximal development; Vygotsky, 1978). In other words, the more the child learns and does, the better the child will be.
Several learning theories drive the child development literature on learning new information and skills. Child development theories on constructivism, neoconstructivism, and neuroconstructivism propose that active learners interact with and draw meaning from their environment based on their current abilities, resulting in a cascading effect of new experiences building on prior experiences (Johnson, 2010; Newcombe, 2011; Westermann et al., 2007). In a similar vein, Dynamic Systems Theory (Thelen & Smith, 1994) proposes an interaction of multiple systems (e.g., motor, visual, auditory), so that even a small improvement in one system can lead to improvements in other systems (e.g., Soska, Adolph, & Johnson, 2010). This literature also includes many examples of scaffolding (and its beneficial effects) that involves caregivers or other environmental support helping children perform tasks step-by-step until the children can perform it on their own (e.g., Obradović, Yousafzai, Finch, & Rasheed, 2016; Vygotsky, 1978; Wood, Bruner, & Ross, 1976), rather than, for example, merely performing the tasks for the children.
3 Cognitive growth across the lifespan
Applying our understanding of strategies to help children learn and grow to help adults do so may trigger cognitive growth in older age groups. Our theoretical approach to lifespan cognitive development (Wu, Rebok, & Lin, 2017) proposes that, across the life course, we transition from learning broadly (i.e., a variety of information and skills) with a great deal of support (e.g., teachers) to learning more specialized information and skills with less support. The approach proposes that this transition leads to less openness to learning new information, which leads to more difficulty adapting to new situations. Reintroducing broad learning with a supportive environment to adults, especially older adults, may lead to cognitive gains. Nguyen et al. (2018) extended this theoretical approach by proposing that the ultimate goal in older adulthood is to maintain functional independence in a changing environment (Golant, 2015; Wahl & Gerstorf, 2018), necessitating adaptation for growth.
The theoretical approach, Cognitive Agility Across the Lifespan via Learning and Attention (CALLA; Wu et al., 2017), proposes that six factors may maximize cognitive growth across the lifespan. Central to CALLA is the proposal that both the quantity and quality of new, broad learning experiences in a supportive environment decline from infancy and childhood to adulthood. Learning broadly (e.g., five varied subjects in school) is expected in younger learners, compared to advanced undergraduates who typically take courses related to their major. After the last year of formal education, adults typically learn skills related to their jobs, incrementally increasing their career skills. Training periods are minimized as much as possible to maximize business productivity and profits. The decline in breadth and intensity of learning experiences across adulthood is a critical, yet overlooked, factor in cognitive aging. CALLA proposes that transitioning across the lifespan from learning broadly to becoming an expert in specific domains can lead to rigidity in relying more on prior knowledge and habits than learning different types of information and skills. This rigidity over decades may lead to functional and cognitive decline in a dynamic environment if the learner does not know what to learn or how to learn new information. The ideas from CALLA are supported in general by research that links cognitively engaged lifestyles with novel and complex activities and careers to a lower risk of cognitive impairment in late life (Andel et al., 2019; Hertzog et al., 2008; Oltmanns et al., 2017; Wilson et al., 2007). Consider this rigidity from earlier in the lifespan: When infants and children are held back from learning, it is considered deprivation (e.g., Nelson, 2007), and significant efforts are made to remove infants and children from the deprived situation and place them in enrichment programs when possible. Yet, deprivation of novel broad learning experiences is normal in aging, particularly after retirement or by the time someone holds a top career position. Perhaps the same broad learning experiences that promote children's growth and development can benefit aging adults.
The CALLA framework proposes that learning how to learn and what to learn across the lifespan includes six factors, which are known to help infant and children. First, infants and children learn via (1) open-minded input-driven learning (e.g., Aslin & Newport, 2012), including learning completely new information via observing patterns in the environment. For example, based on patterns in the environment, the learner can infer whether one event causes another event or that the two events only are associated (e.g., Gopnik et al., 2004). Social and environmental characteristics also matter. In particular, children have responsive teachers who provide (2) individualized scaffolding (e.g., Vygotsky, 1978; Wood et al., 1976). The most effective teachers and mentors model behaviors or skills (e.g., Bandura, 1986) and provide just the amount of support needed by each learner, until they can accomplish the task on their own. Learning is also better in (3) forgiving environments, where learners are encouraged to make and learn from mistakes (e.g., Wong & Lim, 2019). In terms of attitudes and beliefs of the learner, characteristics of learners related to better learning are (4) a serious commitment to learning and (5) a growth mindset/positive self-evaluative beliefs (Barber & Strickland-Hughes, 2019; Dweck, 2006; West & Strickland-Hughes, 2015), believing that they can improve with effort. Learners with a growth mindset, high perceived control beliefs, or strong learning self-efficacy, are able to learn more than their peers who believe their ability is fixed, predetermined, and unchangeable (e.g., Dweck, 2006). Even if the learner does not have a growth mindset, having a mentor who does benefits the learner's progress (e.g., Andersen & Nielsen, 2016). Finally, learning may be faster in the end when (6) learning multiple skills simultaneously, rather than one at a time, because learners could experience more diverse learning problems, examples, and solutions, identify similarities and differences among learning examples, generalize and transfer to novel situations more readily, and engage in more creative problem solving (e.g., Barnett & Ceci, 2002; Gentner, 2005; Rost & McMurray, 2009). For example, learning different subjects at once may strengthen overall understanding of broader topics as they can develop connections between concepts and develop more nuanced and elaborate schemata to evaluate, explore, and understand the world.
The novelty of the CALLA framework rests in examining the context of cognitive aging through the lens of child cognitive development, free from negative stereotypes of aging (e.g., “old dogs can't learn new tricks”). Although the CALLA framework does not capture all aspects of cognitive aging, it captures an important, but overlooked, aspect of growth in older adulthood, which may improve cognitive decline trajectories.
4 Extending CALLA to include adaptation for growth in a dynamic environment
The CALLA framework is comprehensive in explaining learning how to learn and what to learn and why learning, especially broad learning, declines over the life course. However, it does not explain why broad learning should occur throughout the lifespan and what elements are necessary for one to adapt for growth. In this paper, CALLA-X (Fig. 1) extends CALLA to address these issues. Why should broad learning occur throughout the lifespan? As summarized above and incorporated in CALLA-X, the ultimate goal of all learners is to adapt in a dynamic environment to be functionally independent for as long as possible (purple box, see also Nguyen et al., 2018).
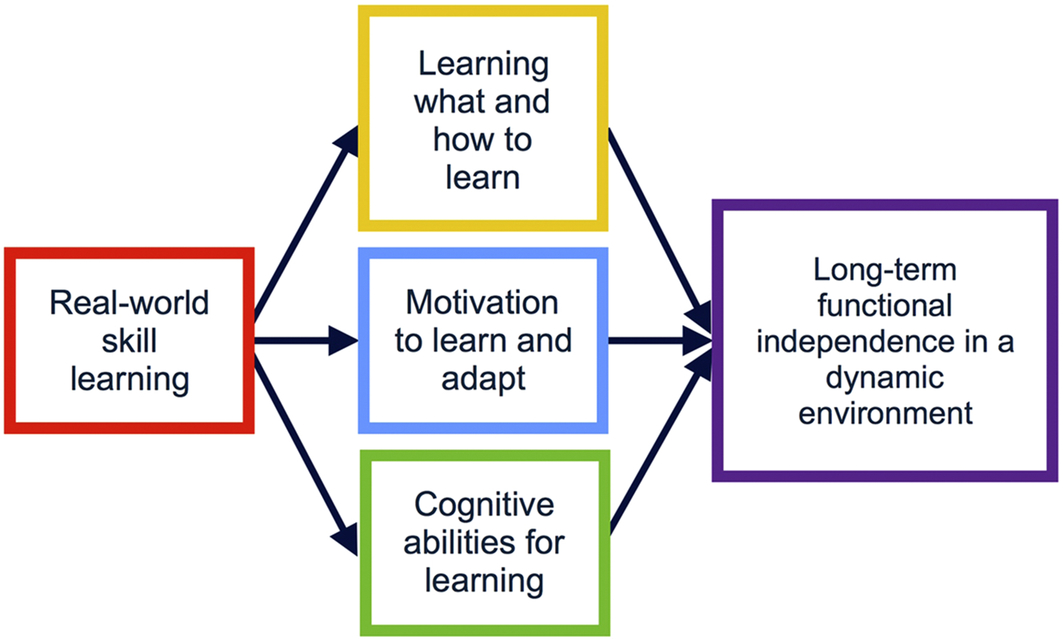
In terms of what elements are necessary for one to adapt for growth, in addition to learning what to learn and how to learn (covered by CALLA, yellow box), there are two additional elements: (1) developing motivation to learn and (2) ensuring adequate cognitive abilities for learning. Although likely an oversimplification, a driving metaphor conceptualizes the roles and interaction of these three elements: Learning what to learn and how to learn is a GPS device, motivation for learning is the fuel, and cognitive abilities for learning is the engine.
Learning what and how to learn: the GPS device. Learning what to learn and how to learn provides a guide to learn relevant information, rather than irrelevant information. It is best to be efficient, yet flexible with what to learn, as the tasks and environment change. In line with factors from CALLA, Wu (2019) notes four ways of learning what to learn, each with their benefits and limitations. First, one can learn from stimulus characteristics (e.g., open-minded input-driven learning from CALLA). These include patterns in the environment, such as frequency or salience of events (e.g., Aslin & Newport, 2012). For adults, learning from patterns in the environment includes determining the appropriate vocabulary to use when repairing a car based on exposure frequency of particular phrases. Infants learn from stimulus characteristics from birth. However, relying only on stimulus characteristics means that the learner may miss important but subtle, or infrequent, information, and be driven haphazardly by the environment.
A second way of learning what to learn is based on reinforcement or feedback (e.g., Balzer & Doherty, 1989). This way is the easiest and fastest way to learn if the learner understands the feedback provided. However, this process is still slow, because every piece of information must be reinforced for learning. For adults, learning from feedback might include learning what causes error messages to pop up on an iPad.
A third way is leaning from people, who may or may not provide feedback (e.g., correcting the learner), but can quickly direct the learner to relevant information (individualized scaffolding from CALLA). Infants begin reliably learning from people by around 6–8 months of age (e.g., Wu, Gopnik, Richardson, & Kirkham, 2011). Prior to that, they tend to learn about people and observe patterns in their behaviors. For adults, learning from people would occur when asking for directions in a foreign country. Learning from people is extremely beneficial as they provide an efficient shortcut to relevant information. However, what is noted as relevant is entirely dependent on the reliability of the instructor.
The fourth way of learning what to learn is based on knowledge acquired from prior experience. Prior knowledge can help the learner determine what is currently relevant or irrelevant based on what was relevant or irrelevant in the past. For example, based on prior knowledge about driving a sedan with an automatic transmission, driving a rental minivan with an automatic transmission is not too difficult. However, if the environment or situation changes and no longer aligns with prior knowledge (e.g., driving on the opposite side of the road in a sports car with a manual transmission), then the knowledge becomes obsolete and may even be misleading. Adaptation is especially necessary when prior knowledge is obsolete.
Following on the idea that learning what to learn depends on prior knowledge, simulated annealing is a computational technique, capitalized by child development research (Gopnik, Griffiths, & Lucas, 2015). The premise of the approach with regard to child development is that learning rate and the overall problem space can be decreased slowly through training and knowledge acquisition from infancy to adulthood (Gopnik et al., 2015). This approach utilizes a complex, but static, environment throughout the lifespan, allowing the learner to stabilize by adulthood. Modeling a static environment assumes that the learning problems encountered across the lifespan are the same, and that younger age groups have to learn the parameters that adults already know (e.g., learning one's native language). Some empirical evidence supports this approach, such as demonstrations that adults are less flexible in generating unique causal inferences than children (e.g., Gopnik et al., 2017), which relates to ideas from CALLA on reduced openness to learning new information with age. The simulated annealing approach also has validity in some cases with slow changes (e.g., learning a native language). However, the approach may not apply to many other situations with faster changes, such as moving to a new country as an adult or learning to use new technological devices as they are invented.
It is not clear how much the amount of learning and the learning rate should actually decrease across the lifespan, given that the environment is dynamic. Reaching targeted solutions and stabilizing over a long period relates to the concept of selectivity, such as developing expertise in a very narrow subject area (Baltes, 1997), which relates to the idea of incremental, narrow learning in CALLA. Selectivity and stabilization can be helpful in a static environment; expertise and canalization of skills may boost performance for well-learned tasks. Moreover, although adaptation may sometimes require incremental learning on existing knowledge structures, the most difficult types of adaptation may require broader learning and building new knowledge structures (e.g., moving to a foreign country).
Considering the annealing metaphor more closely, annealing, as conceptualized in metallurgy, is actually a process that induces malleability to a piece of metal or glass by taking away its brittleness. This conceptualization differs from its conceptualization in child development research, which supposes a narrowed focus and crystallization via cooling and stabilizing over time—i.e., inducing brittleness. The adult learner may resonate more with the metallurgic annealing analogy of removing brittleness because when a piece of metal is formed into a particular structure, its ability to be formed into another structure is limited, due to brittleness. Annealing addresses this issue by inducing malleability to allow further formations, freeing the learner of the brittleness encouraged by prior formations (knowledge). In terms of human abilities, the brittleness from metallurgy aligns with entrenchment in human development, whereas annealing, as defined in metallurgy, aligns with adaptation.
Motivation to learn and adapt: the fuel. Prior studies on cultivating motivation for learning in adulthood draw on child development research (see Wlodkowski & Ginsberg, 2017). Several theories suggest that motivation is key for learning. For example, self-efficacy theories (e.g., Bandura, 1997; Schunk, 1991) emphasizes that individuals' goals and future expectations, as well as their current skills or knowledge (i.e., mastery experiences), drive performance outcomes. Other theories specifically address motivation in young learners, especially in a classroom setting (Deci & Ryan, 1985). Self-determination Theory, with a sub-theory, Cognitive Evaluation Theory, emphasizes social contexts for intrinsic motivation, such as offering choices in tasks rather than rewards. Beyond mastery, social contexts, and internalization (or the process of internalization), a new approach proposes that motivation can be increased from exposure to new information, especially when the learner starts with a low level of knowledge about the content area (Ditta, Strickland-Hughes, Cheung, & Wu, 2019). Extending beyond the classroom, other theoretical and empirical work has emphasized motivation-cognition relationships across adulthood (Braver et al., 2014; Hess, 2014). This work posits that some age-related deficits in cognition are explained by reduced motivation to engage in cognitively demanding activities in late adulthood (Hess, 2014). Interestingly, nontraditional undergraduates (28 + years of age) have been reported to have higher interest and intrinsic motivation than traditional students (younger than 21 years of age), even though the levels of extrinsic motivation do not seem to differ between groups (Bye, Pushkar, & Conway, 2007). These results dovetail with lifelong learning research demonstrating cognitive, health, and well-being benefits for older learners, who likely are high in motivation (e.g., Fernández-Ballesteros, Molina, Schettini, & del Rey, 2012; Hebestreit, 2008). Perhaps these lifelong learners have a growth mindset, a serious commitment to learning, and a forgiving environment to encourage them to maintain their motivation to learn.
Cognitive abilities for learning: the engine. A number of cognitive abilities are required for learning, specifically skill learning, including working memory, cognitive control, and attentional selection (e.g., Meltzer, 2018). Mechanisms for skill learning include both explicit pathways (e.g., learning from feedback) and implicit pathways (e.g., statistical learning). The level of cognitive abilities determines the level of difficulty and complexity in the to-be-learned information and skills. When cognitive abilities are not as high (e.g., in infancy), the environment may provide more compensation and scaffolding (e.g., Obradović et al., 2016). For example, repetition for infants may compensate for their low working memory capacity. Importantly, lower cognitive abilities in infancy compared to young adulthood does not mean that it is not worth encouraging infants to learn skills that are within their reach, and this perspective is relevant across the lifespan.
Empirical tests of the CALLA-X theoretical approach are needed, especially tests that use real-world outcomes. Which aspects of the model most need additional research? The key elements of the model include learning real-world skills, which leads to learning what to learn and how to learn, motivation for learning, and cognitive abilities for learning, which all lead to long-term functional independence in a dynamic environment. We know a great deal about knowledge-based and process-based cognitive trajectories across the lifespan (e.g., Park & Reuter-Lorenz, 2009). Decades of evidence also suggest that we can increase trained cognitive abilities, at least in the short term, via cognitive training interventions (Gross et al., 2012; Kelly et al., 2014; Lampit, Hallock, & Valenzuela, 2014; Morrison & Chein, 2011; Simons et al., 2016; West & Strickland-Hughes, 2015). However, some traditional approaches to training cognitive abilities—which only target specific abilities or strategies or emphasize extended practice (Hindin & Zelinski, 2011; Soveri, Antfolk, Karlsson, Salo, & Laine, 2017; Toril, Reales, & Ballesteros, 2014)—may provide only the metaphorical engine without the GPS navigation system or fuel. In CALLA-X, learning real-world skills, such as playing a musical instrument or learning a new language, is thought to naturally engage all three intermediate elements of CALLA-X. Prior real-world skill learning interventions with older adults, including learning one real-world skill at a time, have found improvements in cognitive abilities that were utilized in the learned skills (e.g., Bugos, Perlstein, McCrae, Brophy, & Bedenbaugh, 2007; Noice & Noice, 2009; Park et al., 2014).
Although we know a fair amount about the development of learning what to learn and how to learn (the GPS device) from infancy to young adulthood, we know much less about these constructs in older adulthood, especially their interactions with motivation to learn (e.g., Hess, 2014; Hastings & West, 2011) and cognitive abilities. These constructs may share similarities across the lifespan in novel learning situations. Novel training programs that target the GPS navigation, as well as the fuel, may be more effective than those that do not (West & Strickland-Hughes, 2015). For example, multifactorial programs, which train additional skills (e.g., attention or relaxation), beyond strategies per se are more effective than those that focus on boosting the cognitive ability alone (Verhaeghen, Marcoen, & Goossens, 1992). Further, results of programs that target meta-cognition (learning what to learn), such as self-monitoring, are promising (Dunlosky et al., 2007), as are those that target the fuel, or individuals' self-evaluative beliefs, such as their memory self-efficacy (West, Bagwell, & Dark-Freudeman, 2008; West & Strickland-Hughes, 2015). Converging evidence also suggests that interventions conducted in groups are far more effective than individual training programs (Gross et al., 2012; Verhaeghen et al., 1992), perhaps due to social benefits to the GPS navigation and the fuel, such as the positive vicarious experiences that bolster self-efficacy (Bandura, 1997).
In the CALLA-X framework, all three intermediate elements—learning what to learn and how to learn, motivation to learn, and cognitive abilities—are important for cognitive and functional growth across the lifespan. Although the three intermediate elements are separable (e.g., one could train each element individually), the CALLA-X approach proposes that until all three elements reach a particular threshold, the learner will be at a disadvantage for the end goal: functional independence via adaptation. In other words, the three elements are part of a system, and removing one element fundamentally changes the system, akin to removing a vital organ. Whether the three elements are separable relates to a key issue in testing the CALLA-X approach: developing control conditions that do not change the system, so that comparisons would then be made between comparable conditions. It is also unclear whether increasing all three elements individually, possibly sequentially, provides the same quantity and quality of beneficial outcomes compared to increasing them simultaneously. Similar to learning studies in child development, studies testing CALLA-X that inherently focus more on gains in older adulthood would push forward cognitive aging research to identify processes and contextual constraints on gains.
5 Building on positive cognitive aging approaches
Baltes introduced several revolutionary ideas, including: (1) there are both gains and losses in older adulthood, but that losses outweigh the gains, (2) the presence of plasticity in aging, (3) that the declines in older adulthood are reversible, and (4) the multidimensionality aspect and individual differences of aging (e.g., Baltes, 1987, 1997). Since the introduction of these ideas in cognitive aging research, more recent studies have investigated the possibility of gains or maintenance in older adulthood, especially in relation to altering unfavorable cognitive decline trajectories (e.g., Cabeza et al., 2018; Hertzog et al., 2008). In particular, many studies aiming to improve cognitive abilities in older adults support the Activity Theory (“use it or lose it”, Havighurst, 1961; Hertzog et al., 2008; Hultsch, Hertzog, Small, & Dixon, 1999). This theory proposes that engagement in frequent and varied intellectually stimulating activities mitigates, delays, or even prevents cognitive decline. Although there is a large literature on increasing cognitive abilities via physical exercise and diet (e.g., Jackson et al., 2016), this paper focuses on cognitive and learning interventions with older adults to lay the foundation for the proposed ideas for future research based on CALLA-X.
Increasing cognitive abilities via cognitive engagement and cognitive training. Hertzog et al. (2008, see also Lindenberger, 2014) emphasize that older adults have upper and lower bounds for cognitive trajectories, and that decline trajectories may be delayed or mitigated via cognitive enrichment. However, it is still unclear what the upper bounds are for a given individual and for the population. An increasing body of research is demonstrating growth in cognitive abilities and neurogenesis later in life (Lazarov, Mattson, Peterson, Pimplikar, & van Praag, 2010; Lövdén et al., 2013; Zatorre, Fields, & Johansen-Berg, 2012, see also Shors, Anderson, Curlik, & Nokia, 2012). They summarize research that shows how formal and informal cognitive activity, in animal models and applied research with humans, can lead to improved cognitive performance in aging. Improved cognitive performance includes short-term gains (e.g., Bugos et al., 2007; Park et al., 2014) or delayed long-term decline or less steep decline in aging (e.g., Rebok et al., 2014).
Some observational studies evaluating the “use it or lose it” claim find that individuals who had cognitively demanding careers or high engagement in lifestyle activities have higher cognitive abilities compared to same-age peers with less cognitive engagement (e.g., Hertzog et al., 2008; Hultsch et al., 1999; Oltmanns et al., 2017; Wilson et al., 2007). Some of this research has demonstrated the benefits of novelty and complexity in work environments (e.g., Oltmanns et al., 2017). Other research aggregates varied activities, such as playing card games with friends and learning a new language, into one general category of “cognitively stimulating activities,” rather than investigating aspects of activities that promote growth versus maintenance (e.g., Hultsch et al., 1999; although see Bielak, 2010; Karp et al., 2006).
Learning new difficult skills, such as a new language, requires building new knowledge structures (e.g., Bugos et al., 2007; Park et al., 2014), compared to playing familiar card games or participating in a well-known activity, which involves incremental learning based on an existing knowledge structure. Indeed, interventions including learning one skill at a time (e.g., Bugos et al., 2007; Noice & Noice, 2009; Park et al., 2014) have found improvements in fluid abilities, such as episodic memory and working memory, perhaps due to building new knowledge structures. For example, Park et al. (2014) showed that learning one skill at a time (i.e., photography or quilting) improved older adults' episodic memory (which relates to remembering events), because the participants had to use episodic memory to learn how to use photography or quilting software and digital cameras or sewing machines.
Building on intervention research including learning one real-world skill at a time, Leanos et al. (2019) implemented an intervention with multiple real-world skills. The intervention focused on teaching participants how to learn and what to learn, while aiming to increase motivation and cognitive abilities, including working memory, episodic memory, and cognitive control. In a feasibility study with six intervention participants in Study 1 and 27 participants in a replication sample (Study 2), Leanos et al. (2019) tested the effects of learning multiple real-world skills simultaneously in older adulthood (60 + years of age). In the intervention, participants were taught what to learn and how to learn in three different domains (creative arts, technology, and language). Their motivation to continue learning new skills anecdotally increased (although future research should include standardized measures for this construct), and the participants' cognitive abilities increased to levels similar to a separate group of middle-aged adults 30 years younger. In terms of testing the impact of skill learning on functional independence, the vast majority of older adults in our intervention were at ceiling from before the start of the intervention on typical functional independence measures (e.g., Instrumental Activities of Daily Living, Lawton & Brody, 1970 and the Everyday Problems Test, Willis & Marsiske, 1993), suggesting that these measures may underestimate functioning in healthy older adults and do not allow for growth (Nguyen et al., 2018). Future research should develop functional independence measures that more accurately capture adaptation for growth in older adults and accommodate rapid changes in the environment, which may require updated measures that are at the pace, or even ahead, of actual changes.
Increasing motivation and self-evaluative beliefs in older adults. Having more negative beliefs about aging earlier in adulthood is related to worse development decades later—including increased rates of heart attack, stroke, and Alzheimer's disease, slower recovery from illness, poorer memory, and even earlier death—even when accounting for health, family history, and other covariates (Levy et al., 2016; Levy, Slade, Kunkel, & Kasl, 2002; Levy, Slade, Murphy, & Gill, 2012; Levy, Zonderman,Slade, & Ferrucci, 2009, 2012). By contrast, research suggests that more positive beliefs can lead to gains or growth, such as through positive subliminal messaging leading to better physical performance (Levy, Pilver, Chung, & Slade, 2014) or through training interventions that improve self-referential beliefs, such as memory self-efficacy (Payne et al., 2012; West et al., 2008; West & Strickland-Hughes, 2015). Evidence suggests that training can positively enhance participants' beliefs about their improvements, as well as their actual performance (Goghar & Lawlor-Savage, 2018; Hastings & West, 2009). This research with older adults mirrors research on the Pygmalion effect in childhood (Rosenthal, 1994)—that beliefs of teachers and parents directly influence cognitive and academic performance in children.
The current approach to cognitive aging research is relatively optimistic: it emphasizes personal control over aging outcomes and recognizes plasticity and benefits of engagement. Despite the optimistic evidence that learning and growth may be possible in older adulthood, cognitive aging research focuses more on maintenance or prevention of decline, rather than growth: The assertion is that atrophy is inevitable in the absence of engagement. Maintenance and preservation are the foundation of many cognitive interventions in aging, particularly those that emphasize extended practice, games, or physical exercise (Boot & Kramer, 2014; Kueider, Parisi, Gross, & Rebok, 2012; Morrison & Chein, 2011; Simons et al., 2016; Strickland-Hughes & West, 2016; Toril et al., 2014). Yet still, some scholars question whether meaningful reductions in decline are possible (Salthouse, 2006).
In line with ideas about maintenance and preservation, cognitive reserve is a concept that relates to how much neural pathology one can acquire before crossing a threshold into functional dependence (Stern, 2009). Cognitive reserve is often measured in older adulthood through years of formal education. Such measurement intrinsically suggests that growth is not possible, or at least not a reliable measure, in older adulthood. Although cognitive reserve theory recognizes individual differences and behavioral influences on cognitive aging, still underlying this theory is the assumption that our goal is to limit inevitable decline, not promote new growth. Another theory that supports maintenance and preservation of cognitive abilities in old age is the Socioemotional Selectivity Theory (Carstensen, 1995, 2006), which states that younger age groups prioritize acquiring knowledge, whereas older populations prioritize socio-emotional gains, such as attending social gatherings with close friends. However, such prioritization later in the lifespan may reflect a resistance to learning and adapting. The few models that focus on learning in older adulthood also tend to highlight compensation for decline. For example, the Scaffolding Theory of Aging and Cognition (Park & Reuter-Lorenz, 2009; Reuter-Lorenz & Park, 2014) focuses on sustained engagement in intellectually challenging activities to develop neural pathways that can help compensate for losses in other neural areas, rather than to learn how to adapt to novel environments. More and more research suggests that there is a great deal of heterogeneity in the amount of gains and losses among older adults: Not every older adult declines, and the amount of decline for those who do varies a great deal. Conducting more research on gains in older adulthood would lead to a better understanding of where the ceiling is for a variety of cognitive profiles (see testing-the-limits idea in Baltes & Kliegl, 1992).
6 Anecdotal evidence on learning new skills in adulthood
In addition to the scientific literature supporting CALLA-X, personal anecdotes also support the framework. Popular media contains several examples of middle-aged and older adults learning new skills. Ernestine Shepherd broke the record for the world's oldest body-builder, but did not start exercising until her 50s. Irving Olson learned how to use cutting-edge photographic techniques as a 101-year-old. Kay Lorraine graduated from law school at 70 years old. At 64 years of age, Diana Nyad swam without a shark cage from Cuba to Florida (110 miles), which she was not able to do earlier in life, at 28 years of age. Memoirs of older adults who learned new skills also exist, such as Late to the Ball (learning tennis as an older adult; Marzorati, 2016) and Old in Art School (receiving a BFA and MFA in painting after retiring as a History professor from Princeton; Painter, 2018).
We also note anecdotes that we gathered from middle-aged and older adults who learned new skills. In these anecdotes, the learners highlight having a better understanding of what to learn and how to learn, increased motivation for learning, and increased cognitive capacities for daily tasks. One woman quit her academic career and went to law school at 45 years old; another started attending gym classes in her mid-50s and is widely known as an unbeatable “cycling machine” by undergraduate students. Perhaps the best example of the CALLA-X model in the wild is from the parents of our graduate student. In 2 years, they went from complete novices to expert beekeepers with six colonies. We asked them about how they learned: they used online social media groups, they watched YouTube videos, they read books, blog articles, and research articles, they shadowed master beekeepers to “volunteer” (learn), and they attended community beekeeping groups. They had to make decisions about what they needed to learn, wrote their own “syllabus” and assigned their own “homework.” They had to source expert teachers in the community. When supports were missing, they even started their own beekeeping group (they are on the board), wherein they go on site and help other people set up and troubleshoot bee colonies. They amazed us with their knowledge. We asked permission to describe this experience in the present paper, and they said yes, and offered to teach us beekeeping. Their story highlights key elements of CALLA-X: they learned what to learn and how to learn, and they were motivated and believed in themselves. Although it is not clear whether their cognitive abilities increased throughout the process, they had to have at least a relatively high level of cognitive abilities to achieve what they did. These anecdotes mirror the more general motivational, health, well-being, and cognitive benefits documented with lifelong learning (e.g., Fernández-Ballesteros et al., 2012; Hebestreit, 2008).
We also gathered testimonies from older adults who participated in the intervention from Leanos et al. (2019). Many noted their increased confidence and motivation to learn, especially with technological devices. Those in the art and music classes noted that they saw and heard things in new ways (open-minded input-driven learning)—that they often would pull over while driving to enjoy the colors of a sunset or some wildflowers on the side of the road. They also created their own learning plans for future learning endeavors, which outlined how they would continue learning. Some noted how inactive they were after retirement, and how easy it was to stay inactive. All noted their determination to continue learning for the sake of learning, as well as learning to adapt to the environment as it changes.
7 Implications and future directions
CALLA-X highlights the importance of the ultimate goal (functional independence in a dynamic environment) and the role of learning, motivation, and cognitive abilities to reach that goal. Below, we highlight three implications that CALLA-X has on existing cognitive aging research.
Developing new interventions including all three intermediate factors. Future research can develop interventions to promote growth via increasing the three intermediate factors: learning what to learn and how to learn, motivation to learn, and cognitive abilities for learning. Studies on increasing fluid cognitive abilities via cognitive training and increasing motivation via increasing self-efficacy are extremely useful, and their use is reflected in CALLA-X. However, without knowing what to learn or how to learn, it may be unclear what is relevant to learn for adaptation. These three intermediate factors may be important to adaptation for growth just as a GPS device, fuel, and engine are for driving with a purpose. Therefore, interventions aiming to increase all three factors may be the most useful for adaptation to promote long-term functional independence in adulthood.
New adaptive assessments for functional independence. CALLA-X proposes that the goal of cognitive interventions should be functional independence in a dynamic environment. Currently, functional independence in healthy older adults is defined by the ability to complete important, but basic, everyday tasks, such as going grocery shopping and doing housework (Instrumental Activities of Daily Living questionnaire, Lawton & Brody, 1970). CALLA-X proposes that functional independence in a dynamic environment necessitates learning new skills as they become relevant for daily life (e.g., accessing online medical records). Therefore, new measures of functional independence could be created and adapted as different skills become necessary for everyday activities.
Focusing on growth to raise the standard for what it means to be a successful/optimal ager. Focusing more on growth instead of maintenance or decline may introduce a new perspective in cognitive aging research. If one assumes growth, then the absence of growth or slower-than-expected growth is seen to be an issue. Indeed, in the child development literature (e.g., Johnson, 2010; Newcombe, 2011; Thelen & Smith, 1994; Vygotsky, 1978; Westermann et al., 2007), growth with support is expected and studied. Expecting growth in cognitive aging research may change study designs, hypotheses, and interpretations of findings (e.g., interpreting maintenance as a type of decline). For older adults themselves, expected decline and other negative attitudes about aging may lead to self-fulfilling prophecies and premature decline, including poorer memory, greater likelihood of disease, slower recovery time, and earlier death (Levy, 2009; Levy et al., 2009, 2016, 2002; Levy, Slade, et al., 2012; Levy, Zonderman, et al., 2012).
In this paper, we raise the standard for cognitive aging from maintenance to the potential for long-term growth in cognitive and functional abilities. Currently, successful and optimal aging includes maintaining cognitive abilities (Depp & Jeste, 2006; Rowe & Kahn, 1997). Even with older adults with better than normal cognitive abilities (i.e., supernormals/superagers), maintenance of high cognitive abilities seems to be the goal (Lin et al., 2017). We leverage research from across the lifespan to highlight how long-term cognitive and functional growth might be possible in older adulthood. Perhaps a new tier of thriving older adults can be created to provide room to grow beyond maintenance of abilities.
Society, and perhaps researchers, may be underestimating the potential for increasing cognitive and functional gains in older adulthood because we may not have implemented optimal strategies for encouraging cognitive and functional growth. Moreover, pervasive negative stereotypes about aging characterize the older person as senile, slow, forgetful, distractible, and demented (e.g., Chasteen, Kang, & Remedios, 2011; Hummert, 2011). Many individuals expect aging to mean an inevitable decline in their memory and cognition (Berry, Hastings, West, Lee, & Cavanaugh, 2010; Dark-Freudeman, West, & Viverito, 2006; Lineweaver, Berger, & Hertzog, 2009). These attitudes are often held by adult learners, policy-makers, educators, and societal stakeholders. Limiting beliefs about one's capabilities, such as age-related declines in self-efficacy or a sense of control (Beaudoin & Desrichard, 2011; Strickland-Hughes, Ebner, Smith, & West, 2017; West et al., 2008) are likely associated with reduced cognitive engagement and lower likelihood of pursuing new broad learning opportunities in adulthood. For example, confidence in one's memory abilities predicted memory performance years later (Valentijn et al., 2006).
What can we do at the societal level to address these concerns? Initial anti-ageism campaigns, such as “This Chair Rocks” (https://thischairrocks.com) and positive aging photo competitions (e.g., Grantmakers in Aging) are promising. Further, the World Health Organization has initiated a global anti-aging campaign in research and policy making (Officer & de la Fuente-Núñez, 2018). Lifelong learning initiatives, such as the Osher Lifelong Learning Institute, Institute for Learning in Retirement, Road Scholar, University of the Third Age (McWilliams & Barrett, 2015; Narushima, Liu, & Diestelkamp, 2018) could be more accessible and better subsidized, or even free, just as how all children are entitled to a free, quality education. Massive Open Online Courses, and other free online learning tools, have made promising headway in offering accessible learning opportunities for learners of all ages (e.g., Torres & Beier, 2018). Perhaps with more accessible learning opportunities, in addition to forgiving, supportive environments, adaptation for growth could be more easily achievable across a variety of demographic backgrounds.
8 Conclusions
Decades of cognitive aging research have brought us to an optimistic view, emphasizing plasticity and interventions for maintenance in aging, rather than the initially pessimistic view where universal, inevitable decline was assumed. Given that older adults will outnumber children by year 2035 (US Census Bureau, 2018), understanding the true potential for adaptation for growth across all of adulthood is critical. Although the current approach to cognitive aging research is relatively optimistic, this optimism can be extended. A theoretical approach (Wu et al., 2017) proposes that triggers for cognitive development (i.e., learning what to learn and how to learn) earlier in the lifespan may benefit learners later in the lifespan. Further, adaption for growth is a common goal across the lifespan because the environment is dynamic (Nguyen et al., 2018).
We combine and extend these approaches by proposing a framework for how and why learners should adapt for growth across the lifespan. The key elements of the model include learning real-world skills, which leads to increased learning what to learn and how to learn, motivation for learning, and cognitive abilities for learning, which all lead to increased long-term functional independence in a dynamic environment. In particular, more research on learning what to learn and how to learn across the lifespan, especially in older adulthood, may yield important insights for achieving and maintaining functional independence in the real world. Raising the standard for cognitive aging from maintenance to learning and growth may lead to the development of future cognitive interventions that enhance cognitive and functional abilities in older adults beyond known limits.
Acknowledgments
We thank Robin West, Emily Mroz, Ilana Bennett, and Cecilia Cheung for helpful comments on prior versions of this manuscript. We also thank George Rebok and Herbert Boeckels for insightful discussions about ideas in this manuscript, and Nathan Martin for proofreading help.