Neural dynamics of visual and semantic object processing
Alex Clarke* Department of Psychology, University of Cambridge, Cambridge, United Kingdom
* Corresponding author: email address: [email protected]
Abstract
Recognizing objects involves the processing of visual properties and the activation of semantic information. This process is known to rely on the ventral visual pathway extending into the anterior and medial temporal lobes. Building on the established neural architecture supporting object recognition, I argue that we need dynamic accounts that can explain the speed of recognition and incorporate feedforward and recurrent processing dynamics. In order to explain recognition, we need explicit models of visual and semantic processing, situated at the level of individual objects, and methods to apply such models to time-resolved neuroimaging data. Here, I outline a computational and cognitive approach to modeling the incremental visual and semantic properties with a neural network, before providing an account of how we access meaning from visual inputs over time. I argue an early phase of processing extracts coarse meaning from visual properties, before long-range recurrent processing dynamics enable the formation of more specific conceptual representations beyond 150 ms. Various sources of evidence underlie the importance of feedback for detailed conceptual representations, with connectivity between the anterior temporal and posterior temporal regions playing a major role. Finally, I will discuss how the nature of the task impacts the processing dynamics, and discuss the role the environmental context could play.
Keywords
Semantic memory; Vision; Object recognition; Dynamics; Time course
1 Introduction
When we see an object, we don't perceive it as a collection of edges, textures, colors and shapes, but as a holistic entity, one that has value, purpose and something we can meaningfully relate to. Accessing meaning from visual inputs is a vital function—it is the way we understand the world we see, and it is not solely a visual problem. Behaving in the world requires that we flexibly access different aspects of meaning, depending on the situation we find ourselves in and the environmental contextual constraints. Appropriate behavioral responses require that we respond quickly and accurately, necessitating that object recognition is fast. Here, I focus on the rapid neural dynamics of human visual object recognition—where recognition critically includes the visual and semantic processing of an object. I argue this is underpinned by rich dynamic interactions within the ventral visual pathway (VVP) over the first half second of seeing an object, culminating in an understanding of what we see.
2 Architecture of object recognition
Non-human primate research, neuropsychology and fMRI in humans have provided unequivocal evidence that the visual processing of objects is dependent on a distributed network of regions throughout the occipital, temporal and parietal lobes (Kravitz, Saleem, Baker, Ungerleider, & Mishkin, 2013). Of particular significance is the VVP along the axis of the occipital and temporal lobes, that acts to transform low-level visual signals into more complex and higher-level visual representations (Bussey, Saksida, & Murray, 2005; Cowell, Bussey, & Saksida, 2010; DiCarlo, Zoccolan, & Rust, 2012; Hubel & Wiesel, 1962; Kravitz et al., 2013; Riesenhuber & Poggio, 1999; Tanaka, 1996). This encompasses a series of cortical transformations from primary visual cortex, through many increasingly complex visual areas in the occipital lobe, to the lateral, ventral and medial aspects of occipito-temporal cortex, in particular the fusiform gyrus, and extends into the ventral and medial anterior temporal lobes. The representations constructed along this pathway are utilized in service of a vast array of cognitive functions such as memory, learning, language and decision making.
Hierarchical visual processing along the VVP is the dominant conception of visual object processing. Early visual regions, such as V1, V2 and V4, process the low-level visual properties of orientation of lines and edges and have small receptive fields (Hubel & Wiesel, 1962; Riesenhuber & Poggio, 1999). As we move to later layers of this hierarchy, the complexity of visual stimuli that drives the neural response, and the receptive field sizes, increase. Further along the VVP increasingly complex visual information is coded, such as complex shapes and parts of objects, as highlighted by the studies of Tanaka and colleagues who determined which stimuli produced the maximal responses from neurons in IT. Across a series of studies, the strongest responses were seen from what they termed “moderately complex” visual stimuli, leading to the claims that neurons in IT are turned to visual shapes that are more complex than low-level regions, but do not comprise whole objects (Kobatake & Tanaka, 1994; Tanaka, 1996; Wang, Tanaka, & Tanifuji, 1996). In contrast, the perirhinal cortex, at the apex of the VVP, codes for the most complex conjunctions of simpler visual information in posterior temporal regions (Bussey et al., 2005; Cowell et al., 2010; Miyashita, Okuno, Tokuyama, Ihara, & Nakajima, 1996; Murray & Bussey, 1999) thus enabling the representation of complete objects.
Such hierarchical models have played a pivotal role in shaping theories of visual object recognition in humans, who show largely similar spatial profiles along the VVP to non-human primates. Much of this research was understandably cast in terms of visual processing, with the implication that the neuronal responses were isolated from higher cognitive functions. However, when we consider human research in object recognition, it becomes more obvious that visual processing is intertwined with our knowledge and experience with these objects, at least at some levels of the VVP. Object recognition is more than the visual processing of a stimulus and cannot be accomplished without access to object semantics.
Research on the semantic representation of objects has tended to focus either on the semantics of superordinate categories (e.g. an animal, a tool, vehicle), or on the semantics of object-specific concepts (e.g. a basic-level concept such as a dog, a hammer, a motorbike). While earlier work had shown that increasingly complex visual information is processed along the VVP, this recent research highlights a parallel progression of increasingly complex semantic information represented in increasingly anterior regions of the VVP (Clarke & Tyler, 2014; Iordan, Greene, Beck, & Fei-Fei, 2015; Tyler et al., 2013). There are widely seen effects of superordinate category knowledge (e.g. knowing an object is an animal, or a tool) in the posterior ventral temporal cortex (pVTC; such as the posterior fusiform gyrus, inferior temporal gyrus and lateral occipital cortex) for variety of categories such as faces, places, body parts, houses, tools and animals (Chao, Haxby, & Martin, 1999; Clarke & Tyler, 2014; Downing, Jiang, Shuman, & Kanwisher, 2001; Epstein, Harris, Stanley, & Kanwisher, 1999; Kanwisher, McDermott, & Chun, 1997; Kriegeskorte et al., 2008; McCarthy, Puce, Gore, & Allison, 1997; Tyler et al., 2013). This suggests that semantic information is represented in a relatively coarse fashion in the pVTC, where objects from different categories can be distinguished, but objects from the same category are not differentiated based on their semantics (Clarke & Tyler, 2014). This last word is important: different visual images can be distinguished based on activity in pVTC but the semantic identity—over and above anything explained by physical image properties—may not be (at least in the initial response).
However, object representations within the VVP must be sufficiently rich and complex to support the recognition of individual objects, not just the category they belong to. This object-specific semantic information is known to be dependent on the perirhinal cortex (PRC) (Clarke & Tyler, 2014; Kivisaari, Tyler, Monsch, & Taylor, 2012; Martin, Douglas, Newsome, Man, & Barense, 2018; Tyler et al., 2013; Wright, Randall, Clarke, & Tyler, 2015). The PRC forms complex semantic representations of objects based on combining simpler information in pVTC and allows for the differentiation between semantically similar items (Barense et al., 2012; Barense, Rogers, Bussey, Saksida, & Graham, 2010; Clarke & Tyler, 2014; Cowell et al., 2010; Kivisaari et al., 2012; Taylor, Moss, Stamatakis, & Tyler, 2006). Importantly, I further claim this fundamental ability to dissociate between semantically similar objects is supported by connectivity between posterior and anterior regions of the VVP, as suggested by connectivity studies (Campo et al., 2013; Clarke, Devereux, & Tyler, 2018; Clarke, Taylor, & Tyler, 2011) and functional imaging of semantic dementia patients (Mummery et al., 1999)—see Section 5.
3 The need for dynamic models
The basic architecture of object recognition seems, therefore, to be broadly understood, highlighting the importance of the relationship between vision and semantics for recognizing objects, and of the roles of pVTC and PRC in object semantics. Yet this view of recognition—where both activity and the complexity of object information progresses along the posterior to anterior axis in the VVP—is fundamentally incomplete in so far as it implies a feedforward and bottom-up model underpinning cortical processing. In contrast, the brain's anatomical structure suggests that complex interactions between bottom-up and top-down processes must be a key part of object processing as demonstrated by the abundance of lateral and feedback anatomical connections within the VVP and beyond (Bullier, 2001; Hegdé & Felleman, 2007; Lamme & Roelfsema, 2000; Wyatte, Jilk, & O'Reilly, 2014). Hierarchical, bottom-up, models of recognition can only capture part of the story (Rajalingham et al., 2018; Serre, Oliva, & Poggio, 2007) and may miss vital cognitive aspects of semantic memory for true object recognition.
In addition to providing an account of object recognition that incorporates recurrent neural dynamics, accounts must also consider the speed of recognition. A compelling series of studies from Thorpe and colleagues have clearly demonstrated that we can identify an object and make rapid decisions within 150 ms. Using a paradigm where two scene images are presented to the left and right of a central fixation, and participants must make a saccade to the image containing the target (e.g. an animal), they have shown that accurate saccades can be made in as little as 120–150 ms (Crouzet, Kirchner, & Thorpe, 2010; Kirchner & Thorpe, 2006). This shows that a target object can be detected and a saccade made in very little time, placing constraints on the nature of processing supporting the decision. However, it must be kept in mind that the task required a decision that a pre-determined item is either on the left or right, and so will involve prior top-down processing to detect the target. Further, it is also often the case that the task involves the distinction between two different kinds of thing (e.g. between an animal and a vehicle). Regardless, these studies clearly highlight that coarse semantic decisions can be made very rapidly—although the precise representational nature of the information driving behavior remains elusive. Turning to a more natural situation associated with object recognition—namely, picture naming—an influential model developed by Levelt and colleagues considered that conceptual access from a visual input occurred within 150–175 ms after picture onset, and the correct lemma is selected within 250 ms (Indefrey & Levelt, 2004; Levelt, Meyer, & Roelofs, 1999; Levelt, Praamstra, Meyer, Helenius, & Salmelin, 1998). However, as indicated by our later discussion, these timings for conceptual and lemma selection may be under-estimates (see Section 5), perhaps reflecting a lower-bound. When considering the timing of specific operations, it is vital to consider the dynamics of what may have already happened, such as visual processing for semantic access of pictures, and situate cognition in a dynamically evolving process. Further, this process will be shaped by many factors about the stimulus itself, the task and the context. Regardless, such studies clearly show that accessing high-level properties from visual inputs could happen very rapidly, and must be underpinned by complex feedforward and recurrent dynamics. Therefore, our accounts of object recognition must incorporate this, rather than being solely dependent on evidence from more temporally static and less dynamic sources, such as fMRI and neuropsychology, alone.
In the following sections, I aim to set out how we dynamically access semantic information from visual inputs, and how this process can be shaped depending on the nature of our goals, and the environment we find ourselves in. The over-riding goal of object recognition is to understand what the object is, which can then dictate how best we can respond. What's imperative here is to (1) access the meaning of an object from the visual input and (2) access the relevant semantic information for the given situation (e.g. the given task or goal). This goes well beyond accessing the label of an image, which is often the goal of computational models of vision, but is insufficient to explain either the temporal evolution of meaningful information from visual inputs or rapid conceptual priming effects (e.g. Friese, Supp, Hipp, Engel, & Gruber, 2012; McPherson & Holcomb, 1999). In this sense, models of vision tackle what has become known as “core” object recognition, although I would argue that recognition must involve accessing some degree of object semantics, rather than being a purely image-based process. In what follows, I look to go beyond core object processing, by explaining how visual inputs dynamically activate meaning, and how meaning can be flexibly accessed through manipulations of the task or the context. What is central in achieving this are appropriate cognitive and computational models of visual and semantic processing, which can provide quantifiable measures for individual objects that can be tested against the brain activity elicited by those objects.
4 Modeling visual and semantic representations of objects
The primary challenge for human cognitive neuroscience is to uncover the relationship between patterns of brain activity and the nature information processing. Studies relying on comparing brain activity between a small number of controlled and balanced conditions has led to a wealth of important findings. However, if we are interested (1) the semantic processing of individual objects—not conditions or groups of objects and (2) understanding multiple facets of those objects across visual and semantic parameters, then we must take an alternative analysis approach. This encompasses (1) using condition-rich designs where every object is considered a condition and (2) multivariate analysis where each object can be characterized through multiple measures that span the visual and semantic domains. This can be achieved using an explicit model of objects and determining to what extent different aspects of the model can explain the observed data—at the level of single objects. Moreover, this seems the relevant level of detail to address questions of object recognition, as we will naturally recognize something as a dog, rather than an animal (Rosch, Mervis, Gray, Johnson, & Boyes-Braem, 1976). Under this approach, bridging the gap between brain and cognition requires we have multi-faceted cognitive and computational models of the world. We are at a point now where we can utilize current advances in computational modeling to obtain quantifiable estimates of the incremental object representations from low-level visual inputs to complex semantic representations.
One component of such an explicit model is currently best captured through convolutional deep neural networks of visual processing. There has been a surge of interest in the use of these deep neural networks (DNNs) for vision, both to provide a highly successful engineering solution to labeling objects within complex images (Krizhevsky, Sutskever, & Hinton, 2012) and to map the outputs from the DNN to brain representations of objects in space (Devereux, Clarke, & Tyler, 2018; Güçlü & Gerven, 2015; Khaligh-Razavi & Kriegeskorte, 2014). DNNs for vision are composed of multiple layers, each containing nodes sensitive to different image features. As the layers progress, the nodes become sensitive to more complex, higher-level image features in a similar progression to the human ventral visual pathway (however the image features the nodes are sensitive to are highly dependent on the training set and task). In this way, DNNs can capture low-to-high level visual properties of images, but they tell us nothing about an object's semantic representation (i.e. while the DNNs can provide accurate labels for images, they do not capture the semantic relationships between objects), while their ability to replicate detailed behavior may also be limited (Rajalingham et al., 2018).
As DNNs advance, and become multi-functional (or multi-cognitive) and more dynamic (e.g. Canziani & Culurciello, 2017), there is no reason why they can't incorporate semantic information about objects, either explicitly coded or learnt through the co-occurrence structures present in the world. As an initial step toward the integration of visual and semantic computational accounts, Devereux, Clarke and Tyler recently combined a DNN for vision with a recurrent neural network for semantic properties (Devereux et al., 2018). Recurrent attractor networks (ANs) were constructed where the activation across the nodes captures the activation of different semantic features of objects (such as “is round,” “has a handle,” “is thrown,” etc.). Recurrent cycles through this network gradually change the input (in this case the input provided by the visual DNN) to express the semantic features associated with that object. The dynamics of how these nodes become activated has been found to mirror both behavioral responses and MEG time courses (Clarke, Taylor, Devereux, Randall, & Tyler, 2013; Randall, Moss, Rodd, Greer, & Tyler, 2004)—where shared features (i.e. features that are shared across many different concepts like has legs of made of metal), reflecting category structure, are activated before distinctive features (i.e. features associated with very few concepts), which, together with shared features, allow a concept to be differentiated from other similar concepts. By using the output of the visual DNN as input into the semantic AN, Devereux et al. provide a potential route by which visual representations can directly activate semantic knowledge. Most importantly, however, combining the DNN and AN produces a quantifiable computational approach to model the incremental visual and semantic properties of objects from low-level vision to high-level semantics and is one that can capture the processing of visual objects in fMRI (Devereux et al., 2018) and MEG (Clarke et al., 2018).
The notion of semantics here is just one of many different ways to quantify semantic information about a concrete concept (e.g. Binder et al., 2016; Mahon & Caramazza, 2011; Martin, 2016; Taylor, Devereux, & Tyler, 2011). Utilizing the notion of semantic features, such as “is round,” “has a handle,” etc., provides a number of advantages, as the similarities between objects can be captured (based on the features they share) and discriminating information additionally captured (the more unique features for that object). Semantic features have a long history with neural networks and PDP models (Cree, McRae, & McNorgan, 1999; Farah & McClelland, 1991; Randall et al., 2004; Rogers & McClelland, 2004; Rumelhart, Hinton, & McClelland, 1986), making them an effective way of modeling semantic information of objects within a visuo-semantic neural network.
5 A dynamic account of object recognition
Here I will highlight how work bringing together ideas of cognitive and computational models of objects semantics and neural dynamics has furthered our understanding of object recognition (also see Clarke, 2015; Clarke & Tyler, 2015). Much of the research discussed builds on theories of cortical dynamics where visual signals undergo an initial feedforward phase of processing as signals propagate along the ventral temporal lobe (Bullier, 2001; Lamme, 2003; Lamme & Roelfsema, 2000; Wyatte et al., 2014), before neighboring regions interact through local recurrent connections and recurrent long-range reverberating interactions occur between cortical regions (Bar et al., 2006; Clarke, 2015; Lamme, 2003; Schendan & Ganis, 2012; Wyatte et al., 2014). Although many phases, or iterations, of recurrent dynamics could occur for different cognitive operations (e.g. Schendan & Ganis, 2012), here I will focus on two broad phases—an early phase of neural dynamics covering the first ~ 150 ms after seeing an object and a later phase beginning after around 150 ms (Fig. 1). I argue that these two phases underlie the critical ability to extract meaning from a visual object under optimal conditions (i.e. for unambiguous objects). Much of the evidence discussed is from human MEG, EEG and intracranial recordings, which give measures from large neural populations. As such, this account is considered at a systems level of description, rather than considering the properties of single neurons.
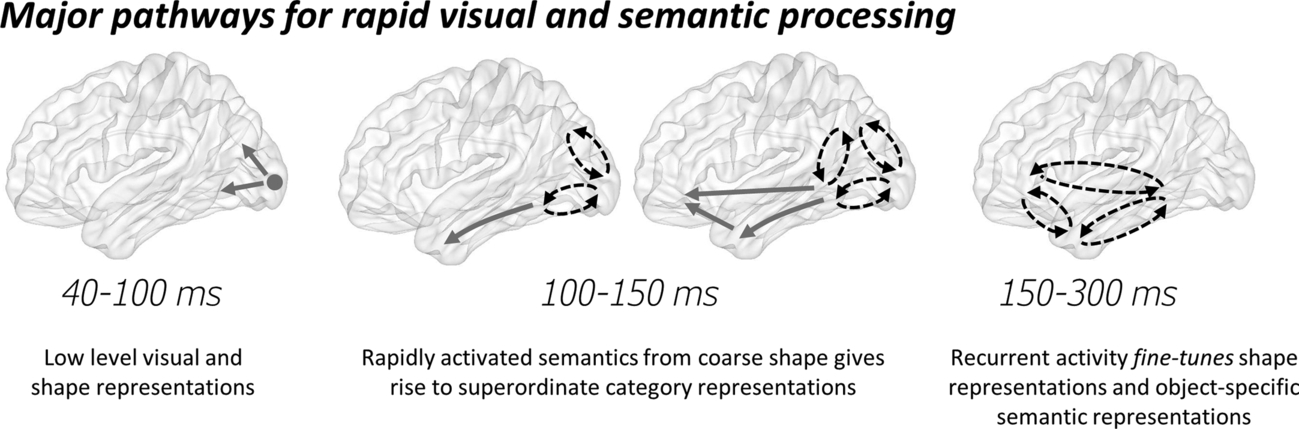
The initial phase of cortical processing after an image appears can be characterized as a feedforward sweep along the ventral temporal lobe during which coarse semantic details are accessed. The initial cortical responses in primary visual cortex are known to show sensitivity to various low-level image statistics, peaking between 50 and 100 ms (Clarke et al., 2013; Martinovic, Gruber, & Muller, 2008; Ramkumar, Jas, Pannasch, Hari, & Parkkonen, 2013; Scholte, Ghebreab, Waldorp, Smeulders, & Lamme, 2009; Tarkiainen, Cornelissen, & Salmelin, 2002), which can be well modeled by computational models of V1/V2 (Bankson, Hebart, Groen, & Baker, 2018; Cichy, Khosla, Pantazis, Torralba, & Oliva, 2016; Clarke, Devereux, Randall, & Tyler, 2015; Clarke et al., 2018). As visual signals propagate forward, they reflect more complex image-based representations (Cichy et al., 2016; Clarke et al., 2018; Seeliger et al., 2017) which also show some invariance to image changes (Isik, Meyers, Leibo, & Poggio, 2014). Moreover, the feedforward progression of signals from the occipital lobe along the posterior temporal lobe that underlies these increasingly complex object representations may be coordinated through feedforward alpha activity (Jensen, Gips, Bergmann, & Bonnefond, 2014; Zumer, Scheeringa, Schoffelen, Norris, & Jensen, 2014). This is supported by an MEG study from Clarke et al. (2018) who showed that alpha activity patterns from regions along the ventral stream related to information from a visual DNN. Using representational similarity analysis (RSA; Nili et al., 2014), they were able to test whether the patterns of brain activity from different regions related to the patterns of activity in different layers of the DNN, finding that information in later layers of the DNN were seen at later times along the VVP. Further, this information match was maximal in alpha activity. Moreover, by combining RSA with functional connectivity, they showed that the visual information from the DNN spread through feedforward connectivity in the VVP, extending into the anterior temporal lobes.
Several studies have now reported activity in the human anterior temporal lobes that is sensitive to semantic dimensions within the first 200 ms of seeing an object (Barbeau et al., 2008; Chan et al., 2011; Clarke et al., 2013; Kreiman, Koch, & Fried, 2000; Mollo, Cornelissen, Millman, Ellis, & Jefferies, 2017). One approach to understand what this activity reflects, in a cognitive sense, is to have an explicit model of object semantics to test against the data, and to do this at the level of individual objects. Clarke et al. (2013) reported that activity in the anterior temporal lobe within the first 150 ms of seeing an object was sensitive to the presence of shared semantic features—so this gives an indication of what kind of thing the object is, suggesting coarse semantic information had been activated. In support of this, Clarke et al. (2015) further showed that a semantic feature model could be used to decode MEG signals at the superordinate category level prior to 150 ms—again suggesting a coarse level of semantic information has been established very rapidly. However, these semantic representations were insufficient to provide basic-level semantic information, which could only be decoded from the MEG signals after approximately 150 ms. This is further supported by additional EEG and MEG work demonstrating concept-level representations become present around this time (Bankson et al., 2018; Schendan & Maher, 2009). In agreement with the general notion of coarse-to-fine processing of visual inputs (Hegdé, 2008; Hochstein & Ahissar, 2002; Trapp & Bar, 2015), this further suggests semantic representations follow a parallel temporal trajectory.
Coarse-to-fine semantic processing is also indicated through behavioral and computational modeling work. Using a go–nogo paradigm, Mace, Joubert, Nespoulous, and Fabre-Thorpe (2009) showed that performance gets slower when the stimulus set contains semantically confusable objects (e.g. a bear being included when the target is a dog) compared to when the set contains very different items. Further, using rapidly presented scene images, Fei-Fei, Iyer, Koch, and Perona (2007) illustrated that general details of the items and scene are produced when images are shown for shorter durations. Both these studies, in addition to the above-discussed MEG data, point to more general semantic details being accessed prior to more complex conceptual object information. Computational modeling studies further support this notion. One neural network model, where the nodes reflect semantic features, shows that shared semantic features (those providing superordinate category information) are activated faster than more distinctive features (that are needed in addition to the shared features for object identification) (Devereux et al., 2018; Randall et al., 2004), while it is also acknowledged that coarse semantic decisions can be faster than basic-level decisions if the neural network has time constraints (Rogers & Patterson, 2007). Overall, neural, computational and behavioral evidence points to a coarse-to-fine semantic trajectory during visual object recognition.
Beyond an initial largely feedforward phase of processing (although local recurrence will occur that is distinct from long-range recurrence; Lamme, 2003; Wyatte et al., 2014), long-range recurrent activity is thought to take place (Bullier, 2001; Chan et al., 2011; Clarke, 2015; Clarke & Tyler, 2015; Goddard, Carlson, Dermody, & Woolgar, 2016; Lamme, 2003; Lamme & Roelfsema, 2000; Schendan & Ganis, 2012), which may support the formation of more detailed semantic representations. In addition to a computational model of vision, Clarke et al. (2018) further investigated how well activation from a combined visuo-semantic neural network model related to dynamic patterns of brain activity. They showed that semantic features associated with specific basic-level objects related to theta activity in the left anterior temporal lobe and left pVTC peaking around 250 ms. This suggests that object-specific semantic object information is underpinned by recurrent processing dynamics between the left anterior temporal and pVTC. Finally, Clarke et al. (2018) asked the unique question of what dynamics support the transition between visual object information and semantic information. Combining functional connectivity with information time-series (provided by RSA), they showed that visual information could help predict future semantic information in a feedforward direction, and through bilateral feedback from the anterior temporal lobes to the pVTC. This suggests that feedforward and feedback connectivity in the ventral stream supports the transition between visual and semantic object representations within the first half second.
One notion that is clear from this account, is that feedback from the anterior temporal lobe is important for basic-level semantic knowledge. While fMRI research suggests the PRC might be the critical sight for directing feedback (Clarke & Tyler, 2014), research combing neuropsychology and functional imaging more directly supports this. Semantic dementia patients with atrophy centered on the anterior temporal lobes show reduced functional activity in regions posterior to the lesion along the ventral stream, suggesting there is a reduction in feedback activity as a consequence of anterior temporal lobe lesions (Mummery et al., 1999). Further, a large-scale study using MEG and patients with small lesions to the temporal pole and rhinal cortices (perirhinal and entrorhinal), display dysfunctional network activity in the VVP, specifically reduced feedback connectivity from the anterior temporal lobes to the posterior fusiform (Campo et al., 2013). Together, these studies strongly suggest that feedback from the anterior temporal lobes, and from PRC, to the posterior ventral stream constitutes a necessary mechanism for accessing semantically specific object representations.
Overall, research using time-resolved imaging methods have shown that both feedforward and recurrent dynamics in the VVP underpin object representations, where visual inputs activate coarse semantic information within the first 150 ms, and object-specific semantic representations emerge beyond 200 ms supported by recurrent activity, specifically between the ATL and pVTC (Bankson et al., 2018; Chan et al., 2011; Clarke, 2015; Clarke & Tyler, 2015; Federmeier & Laszlo, 2009; Kutas & Federmeier, 2011; Mollo et al., 2017; Poch et al., 2015; Schendan & Maher, 2009).
6 Flexibility of meaning
The account outlined above deals with object recognition as the access of basic-level object information. Like the vast majority of research into object recognition, these studies rely on presenting simple images of objects to people, and recording the neural response to those objects, as one object is presented after another in a long sequence. This presents an un-natural situation (over and above being in a psychology experiment), as the objects presented are unrelated to each other in sequence and devoid of a surrounding context. In reality, we find ourselves in a certain setting or environment, and certain objects are more or less expected—walking down the street, we are likely to see a car, but unlikely to see a tiger. This has the important consequence that when we actually see and recognize an object in the world, our neural processes are already biased by the preceding spatio-temporal context. As such, many of our studies into object recognition do not operate in the manner in which we see the world. A second issue is that what we ask people to do critically changes the neural dynamics of the recognition process. As mentioned earlier, the rapid latencies of eye movements are clearly influenced by the task goals, and so the dynamic process of recognition will be influenced by the task, the surrounding spatio-temporal context, as well as the nature of the input.
In addition to the account described above, I will now look at two cases of how these foundational dynamics are modulated: (1) how the task changes the neural dynamics of the semantic processing of objects and (2) how the environmental context biases recognition.
6.1 Impact of the semantic task
When we see a rabbit, we can think of it as a living thing, an animal, pet or a rabbit, and these are often the kinds of task we use in different studies. Further, identifying an object in different ways will be differentially dependent on different kinds of semantic information. From the perspective of a feature-based model of conceptual knowledge (e.g. Taylor et al., 2011), different features can underlie recognition at different levels of specificity. As mentioned earlier, a concept's shared features (e.g. has four legs, has fur, two eyes, are features of a tiger, but also shared with many animals) will indicate the superordinate category the object belongs to (so animals will likely have the features, four legs, fur, a tail, eyes, etc.) but are unlikely to convey enough information to say what kind of animal it is. This has the consequence that having many shared features leads to quicker superordinate category decisions (Taylor, Devereux, Acres, Randall, & Tyler, 2012), and as animals tend to have more shared features than tools (and other non-living things), animals in general will allow quicker superordinate category decisions compared to non-living things (note that having many shared features relates to the idea of conceptual typicality (Patterson, 2007), although here different aspects of correlational structure are shown to have different effects). However, different properties of semantic features explain basic-level naming latencies, for which non-living things tend to be named faster than animals. The activation of distinctive features is critical for naming objects at the basic-level, and the more correlated the more distinctive features are with the concept's other features, the faster they will activate and the quicker basic-level naming will be. Non-living items, like tools, tend to have distinctive features that are correlated with other properties of the concept (for example, form–function relationships) and so this explains why tools typically have quicker naming latencies than animals. These dissociable effects of conceptual structure were elegantly demonstrated in Taylor et al. (2012) who showed that different semantic properties related to domain and basic-level decisions for objects, highlighting that the way in which we recognize an object impacts the kinds of semantic information that we access.
Accessing semantic information about objects at different levels of specificity is also known to modulate activity in the ventral visual pathway. Using fMRI, Tyler et al. (2004) showed that domain decisions were associated with activity in occipital and posterior VTC, while basic-level naming additionally engaged the anterior-medial temporal lobes, which were argued to be crucial for dissociating between semantically similar objects. This was more directly shown in a further study where Tyler et al. (2013) explicitly demonstrated that activity in the PRC during basic-level naming increased as the more distinctive properties of the concept became less correlated—i.e. basic-level access became more difficult as the distinctive properties were weakly correlated with the other properties of the concept.
Further support comes from Clarke and Tyler (2014) who demonstrated a similar principle using the notion of semantic space—where items with many neighbors in this space would be more difficult to differentiate. Clarke and Tyler (2014) showed that the PRC increased its response during the basic-level naming of objects in more dense areas of semantic space. Further to showing that different kinds of semantic properties are important for different kinds of object recognition tasks, these studies clearly demonstrate that activity within the VVP is modulated by recognizing objects in different ways, and the modulation of activity relates specifically to the semantic properties of that object.
However, these studies tell us little about the modulation of the dynamic neural activity within the VVP. To address this, Clarke et al. (2011) conducted an MEG study where participants recognized the same visual objects in both a basic-level naming task, and a domain decision task. They found no task differences in activity during the first ~ 150 ms, which could imply an equivalent early stage of processing of shared semantic information (required for both tasks) during a predominantly feedforward stage of visual object processing (in line with the above account). However, after approximately 150 ms, enhanced activity was seen during basic-level naming—the task that required accessing both the shared features (to know broadly what kind of object it is) and integrating this with distinctive information (to differentiate it from similar concepts). This enhanced activity was seen in the anterior temporal lobe before the posterior temporal lobe and was seen alongside increased beta/gamma functional connectivity between the left anterior temporal lobe and posterior fusiform. The timing of this effect, after ~ 150 ms, and the anterior to posterior propagation of signals is consistent with the idea that recurrent processes within the VVP are modulated by nature of semantic information that is required by the task. This further suggests that while feedforward mechanisms can primarily support accessing coarse semantic information, recurrent dynamics are increasingly needed for accessing detailed basic-level semantic information. Increases in activity in posterior and anterior temporal lobes beyond 150 ms were also observed by Mollo et al. (2017), who further showed these effects in beta band power, further highlighting that the early neural dynamics during semantic processing are modulated by the requirements of the task.
Overall, the current research points to a critical computational role for the human PRC in the individuation of semantically confusable concepts—a role that is not relevant for all semantic distinctions, but only for those requiring highly differentiated representations; such as distinctions between a lion, leopard and cheetah, and moreover highlights that activity within the VVP during objects recognition is flexibly dependent on the goals and nature of the input.
6.2 Situating objects in the world
As we have established, recognizing visual objects depends on dynamic transformations of information from vision to semantics—but in the real world, our understanding of what we see is not solely dependent on interactions between vision and semantics; the environmental context plays a key role. Understanding the extent to which the environment affects the recognition of objects, and the neural mechanisms involved, is an important step because the environment creates expectations about what we are likely to see next. It is well known that such expectations generated by a visual scene can influence object recognition speed and accuracy (Bar, 2004; Davenport & Potter, 2004; Greene, Botros, Beck, & Fei-Fei, 2015; Oliva & Torralba, 2007; Palmer, 1975), and so the dynamics of the visual and semantic processing of an object must also be modulated.
Like object recognition, visual scene analysis is fast (Cichy, Khosla, Pantazis, & Oliva, 2017; Harel, Groen, Kravitz, Deouell, & Baker, 2016; Joubert, Rousselet, Fize, & Fabre-Thorpe, 2007; Kveraga et al., 2011), and follows a coarse-to-fine trajectory (Fei-Fei et al., 2007; Peyrin et al., 2010) where gross categorizations can be made prior to more specific ones (Rousselet, Joubert, & Fabre-Thorpe, 2005). Visual scene perception engages a partially overlapping set of regions compared to object recognition, including the parahippocampal gyrus (PHG), lingual gyrus, retrosplenial cortex (RSC), fusiform gyrus, transverse occipital sulcus, inferior parietal lobe and medial prefrontal cortex (Baldassano, Esteva, Fei-Fei, & Beck, 2016; Bar & Aminoff, 2003; Clarke, Pell, Ranganath, & Tyler, 2016; Epstein et al., 1999; Kravitz, Saleem, Baker, & Mishkin, 2011; Kumar, Federmeier, Fei-Fei, & Beck, 2017; Ranganath & Ritchey, 2012; Stansbury, Naselaris, & Gallant, 2013). In terms of understanding how we recognize objects in the world from the time we first fixate on the item, it is essential that we consider the consequences our perception of a preceding visual scene have for this subsequent object recognition.
The perception of visual scenes will lead to expectations about what objects could appear next in that environment, and as noted, some are more likely than others. Following on our example, being in a city street will create expectations that we see city-related objects such as a car or bus, but not objects normally found elsewhere, such as a lawnmower or tiger. Previous research, principally in the perceptual-decision making and language fields, shows neural responses are modulated by expected events both before, and during the event. Using MEG, de Lange, Rahnev, Donner, and Lau (2013) showed that motor activity before the stimulus, but after a predictive cue, reflected the expected direction of motion in the subsequent stimulus, and this pre-stimulus activity facilitated behavior. This shows that our perceptual expectations can bias activity once expectations are established, and neural signals are not dependent on bottom-up stimulus inputs alone (Kok, Failing, & de Lange, 2014). Expectation continues to modulate activity after the stimulus. Many studies have shown that activity is reduced when the visual stimuli are expected compared to that seen for unexpected stimuli (Alink, Schwiedrzik, Kohler, Singer, & Muckli, 2010; Kok et al., 2014; Kok, Jehee, & de Lange, 2012; Rahnev, Lau, & Lange, 2011). In tandem with reduced activity, stimulus-specific information can be better decoded when the stimulus is predictable and perceptual expectations create a sharper representation of the stimuli (Kok et al., 2012).
These effects are interpreted as showing that our perceptual expectations, or predictions about the world, result in brain states about what is probable or likely to occur next (Summerfield & de Lange, 2014; Summerfield & Egner, 2009; Trapp & Bar, 2015). These top-down predictions facilitate perception, making inferences about objects faster and more efficient when they appear in typical environments (Bar, 2004), consistent with a predictive coding account of perception (Friston, 2005). A central tenant of predictive coding, is that the brain predicts incoming sensory signals based on the previous context. Higher-level regions generate predictions which are communicated to lower regions through feedback connectivity, while feedforward connectivity after the stimulus transmits a signal of the mismatch between the prediction and the sensory input (prediction error). This is supported by fMRI connectivity studies that show the modulation of both feedforward and feedback connectivity during perceptual expectations (Rahnev et al., 2011; Summerfield et al., 2006), although these studies could not temporally dissociate top-down and bottom-up connectivity. It is clear expectations modulate neural processes, however much of this work is based on creating predictable associations between a cue and a simple visual stimulus (e.g. a visual grating). What is less clear is how the complex spatio-temporal dynamics during object recognition is modulated by prior expectations.
In relation to how visual scenes create expectations about objects, the most comprehensive account is provided by Bar and colleagues (Aminoff, Gronau, & Bar, 2007; Bar, 2004; Bar & Aminoff, 2003; Kveraga et al., 2011; Trapp & Bar, 2015). They argue that the PHG and RSC rapidly code for the global scene context, with the medial PFC and orbitofrontal cortex generating predictions about the likely objects in the environment. These predictions impact bottom-up visual object representations through rapid connectivity between the OFC, PHG, RSC and visual regions (Kveraga et al., 2011; also see Brandman & Peelen, 2017), thus facilitating object recognition. However, this account, and most of the evidence in relation to it, makes the core assumption that object recognition occurs at broadly the same time as scene recognition, using paradigms where objects and scenes are presented simultaneously (but see Palmer, 1975). The same can be said for studies testing how objects and scenes interact, as they predominantly assume simultaneous perception. But this does not reflect our default mode of experiencing the world. Our environment is relatively stable over time, while objects can come and go. Typically, we are in an environmental context and then experience or interact with an object. We walk down the street, then turn a corner where we see new objects. Here the context will constrain the objects we are likely to see, and will act prior to seeing the object. This represents an important departure from most studies of visual scene context and object recognition. The important unresolved questions then become, what kind of predictions or constraints does the environment provide, and how do these prior constraints affect the subsequent neural dynamics of visual and semantic processes.
7 Concluding statement
Object recognition, as I have discussed it, is not just a visual process, with visual and semantic object properties dynamically interacting as information evolves over time. It is the semantic properties of objects that enable us to understand and act appropriately, and it is clear that different forms of semantic information—able to drive different behaviors—are accessed dynamically over time. But recognition is also shaped by our current goals and our environment. Our knowledge of where we are in the world biases how we see things in it, and the dynamic processes of recognition will also be biased by this. Future models of objects recognition must be grounded in the world to really explain how we understand what we see, and how we act in specific ways.