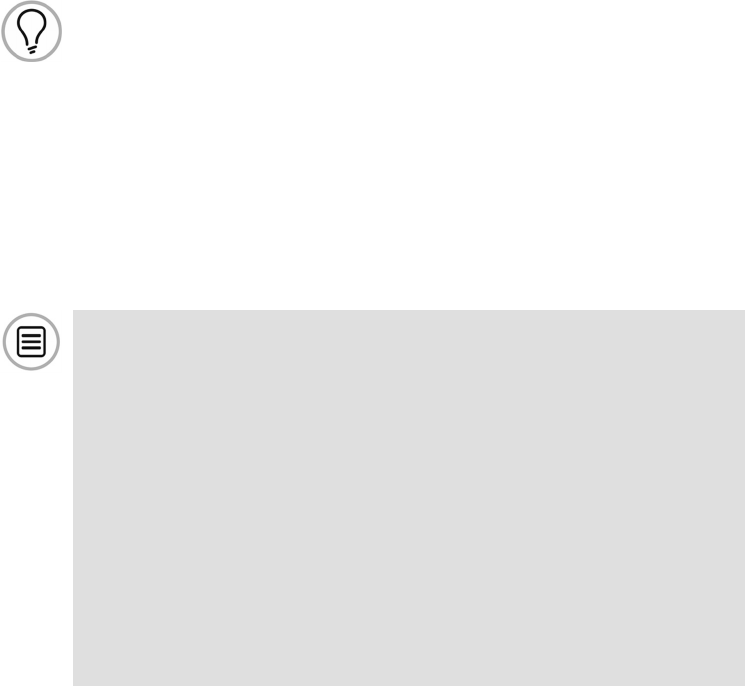
Data, Data Quality, and Descriptive Statistics ◾ 13
for drawing conclusions. We can do with 14, keeping in mind that there could be
small but tolerable errors in our judgment.
Two statistics are of signicant consequence—the mean value is 10.414 and
the maximum value is 30. We are going to apply business rules to evaluate these
statistics and not statistical rules. e mean value of variance, when the estimation
process is mature, should be close to zero. e ideal behavior of estimation errors is
like that of measurement errors; both should be symmetrically distributed with the
center at zero. After all, estimation is also a measurement. e current mean vari-
ance of 10.414 is high, suggesting that the project consistently loses approximately
10% of manpower. is is what Juran called chronic waste.
e second problem is that the maximum value of variance stretches as far as
30%. is is not terribly bad, from a practical angle. Projects have reported much
higher extremities going once in a while as far as 80%. is is anyway a less serious
problem than the mean value.
Both kurtosis and skewness are not alarming.
e median stays closer to the mean, as expected.
ere is no clear mode in the data.
e range is 33, but the standard deviation is approximately 8.5, suggesting
a mathematical process width of six times standard deviation, equal to 51. e
mathematical model predicts larger variation of process. However, even this larger
forecast is not alarming as the mean value.
Overall, the project team has a reasonable discipline in complying with plans,
indicated by acceptable range. e estimation process requires improvement, and it
looks as if the estimation process could be ne-tuned to achieve a mean error of zero.
Box 1.3 SMall IS BIG
e maintenance projects had to deal with 20,000 bugs every week pouring
in from globally located customer service centers. e product was huge, and
multiple updates happened every month and delivered to dierent users in
dierent parts of the world. e maintenance engineers were busy xing the
bugs and had no inclination to look at and learn from maintenance data. e
very thought of a database with millions of data points deterred them from
taking a dip into the data. Managers were helpless in this regard because they
had no case to persuade people to take large chunks of time and pore over
data. Data were unpopular until people came to know about ve-point sum-
maries. A month’s data can be reduced to Tukey’s ve statistics: minimum,
rst quartile, median, third quartile, and maximum. People found it very
easy at merely ve statistics to understand a month’s performance.